Omics codeathon is an established event where life scientists work on research projects. It is held twice a year and led by Olaitan I. Awe (Ph.D.), the current training officer for the African Society for Bioinformatics and Computational Biology (ASBCB).
The April 2024 omics codeathon was virtual.
The primary aim of the omics codeathon is to use omic sequences and bioinformatics to advance the understanding of the biology of model organisms and pathogens to ultimately improve human health. Our research projects typically use human, cellular, cancer and pathogen genomics to investigate the molecular mechanisms of mendelian disorders and complex traits. By using different omics approaches, we are interested in trying to understand how diseases work at the molecular level.
Codeathon participants and applicants come from across the globe, including South Africa, Kenya, Nigeria, Libya, Tunisia, Algeria, Zimbabwe, Morocco, Egypt, Senegal, Mali, Ghana, Uganda, Mozambique, Tanzania, Botswana, Burkina Faso, Gambia, Benin Republic, Ethiopia, Sudan, Malawi, Zambia, Cameroon, Conakry-Guinea, Democratic Republic of Congo, Namibia, Denmark, Malaysia, Turkey, Indonesia, Pakistan, Portugal, Brazil, Poland, Bangladesh, South Korea, Saudi Arabia, Dubai / United Arab Emirates, India, Taiwan, China, Sweden, Finland, Germany, France, Ireland, Spain, Australia, Mexico, United States, United Kingdom and Canada.
The April 2024 codeathon projects were in these categories; Bulk Transcriptomics, Pathogen Genomics, Metagenomics, Human Genomic Variation, Pipeline Development, Biomarker Discovery, Cheminformatics, Clinical Applications, Drug and Vaccine Design, Drug Resistance, Population Genomics, Genome Wide Association Studies, Polygenic Risk Scores, Mendelian Randomisation, Oncology, Structural Bioinformatics, Predictive Modelling, DNA Methylation and Machine Learning.
Omics Codeathon Supporters:
- BioData Sage
- EMBL’s European Bioinformatics Institute
Projects
Title
Team
Project Description
Uncovering Genetic Variations in CAPN10 Gene: An In-silico SNP Analysis
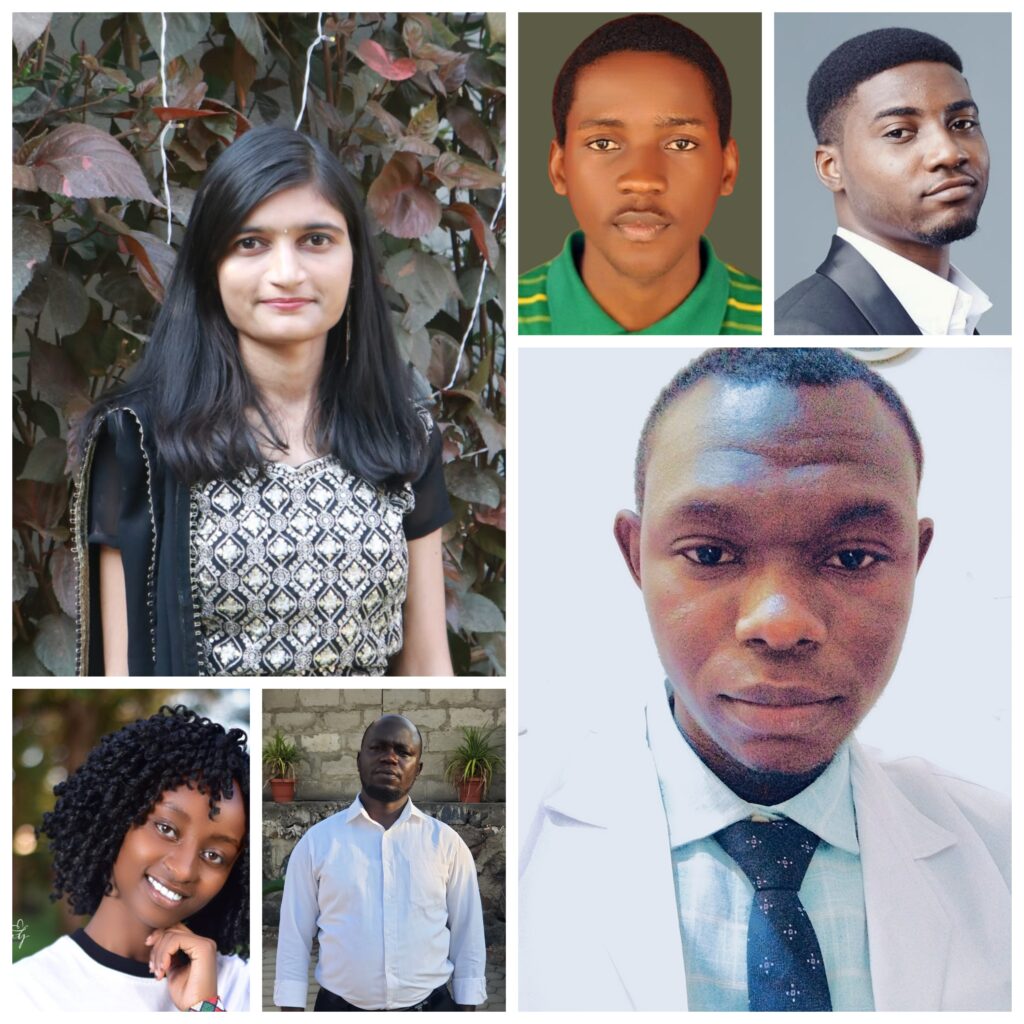
Shivani V. Pawar, Gershom A. Olajire, Nigel Dolling, Purity Njenga, Emmanuel Baluku, and Musa Muhammad Shamsudeen
Our project aims to explore the functional significance of non-synonymous single nucleotide polymorphisms (nsSNPs) within the CAPN10 gene, an important exploration of the complex genetic landscape of type 2 diabetes mellitus (T2DM) susceptibility. By employing a range of bioinformatics tools, we identified 15 nsSNPs likely to exert deleterious effects, with 5 certain amino acid substitutions showing particular promise due to their presence in highly conserved regions and impact on protein stability. This study provides valuable insights into CAPN10 variants, a significant step towards understanding the complex interactions between genetic variation and illness risk. This study therefore facilitates future large-scale population studies and personalized medicine approaches, and potentially informs novel drug discovery efforts.
rhinotypeR: An R package for Rhinovirus Genotyping
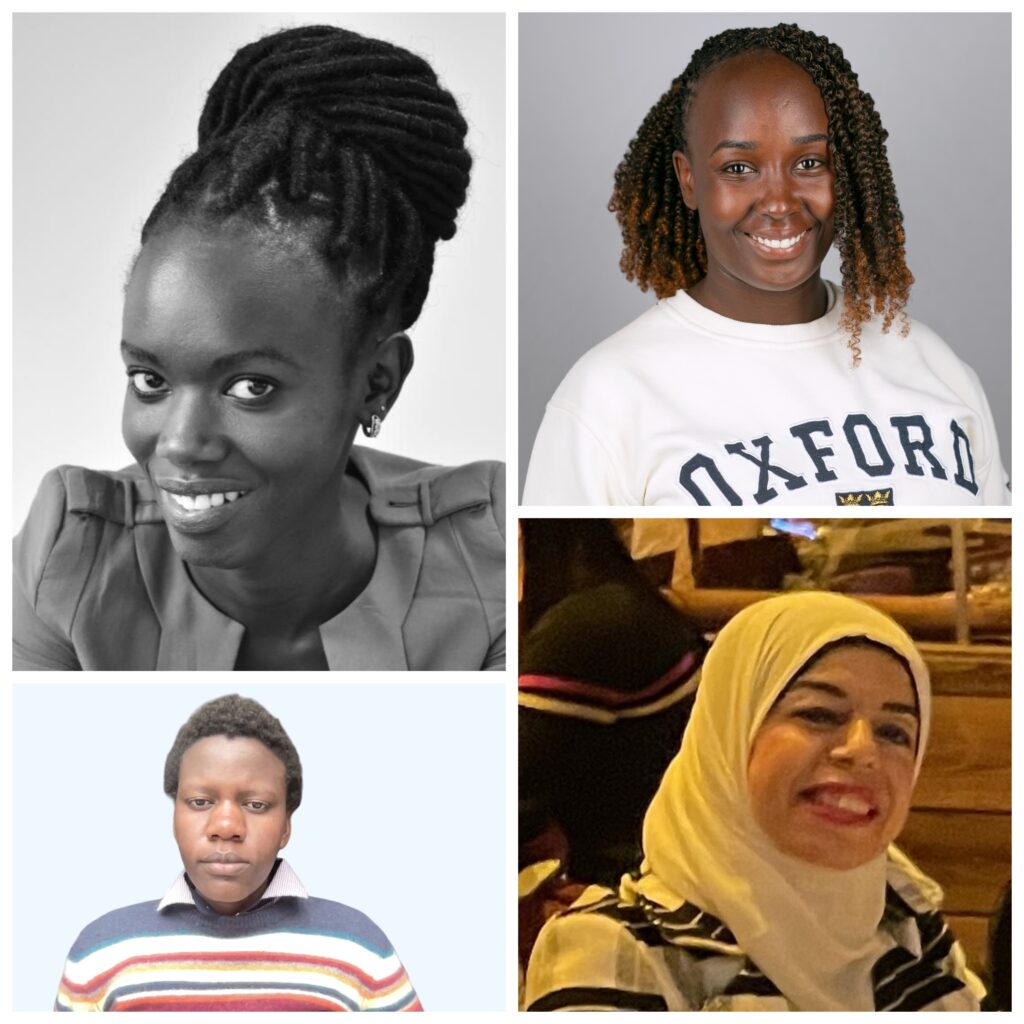
Martha Luka, Ruth Nanjala, Wafaa Rashed and Winfred Gatua
Rhinoviruses (RV), common respiratory pathogens, are positive-sense, single-stranded RNA viruses characterized by a high antigenic diversity and mutation rate. With their genome approximately 7.2 kb in length, RVs exhibit mutation rates between 10^-3 and 10^-5 mutations per nucleotide per replication event. These viruses are classified into 169 types across three species: RV-A, RV-B, and RV-C. Genotype assignment, a critical aspect of RV research, is based on pairwise genetic distances and phylogenetic clustering with prototype strains, a process currently executed through multiple steps, making it laborious. In this study, we developed an R package to streamline RV genotype assignment, thereby facilitating genomic scientists in efficiently genotyping RV infections.
RareInsight: a collaborative rare disease report generator empowering clinicians and patients
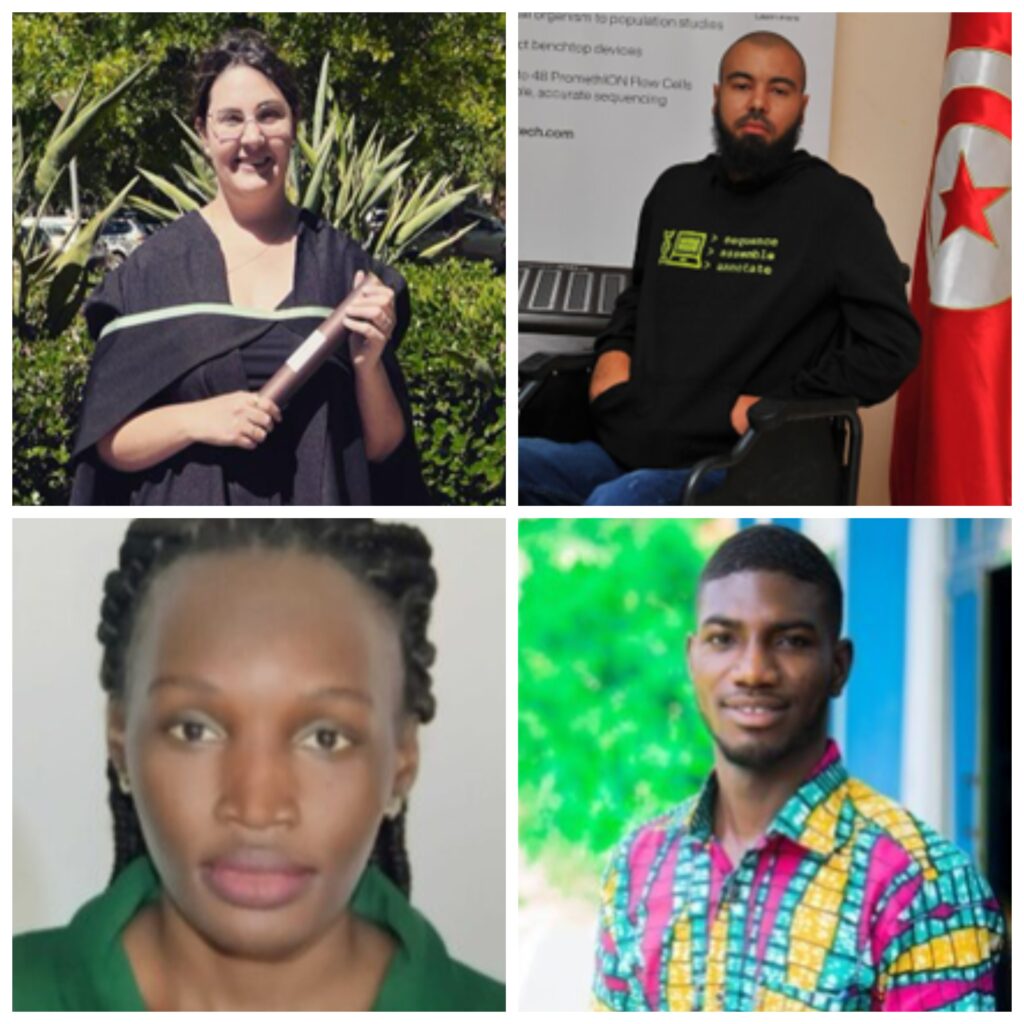
Kimberly Christine Coetzer, Firas Zemzem, Eva Akurut and Gideon Akuamoah
RareInsight is a project aimed at tackling the challenges posed by rare diseases through collaborative efforts in research. Developed as an open-source, interactive dashboard tailored for both clinicians and patients, RareInsight focuses on transforming genetic variant data interpretation.
The project’s core objective is to simplify the complex process of diagnosing rare diseases and improving therapeutic outcomes. RareInsight processes genetic variant data into customizable, interactive reports, ideally generated by nf-core’s rare disease pipeline. These reports are derived from whole genome or whole exome sequencing data and offer advanced filtering options, and diverse export formats.
Developed using ShinyDashboard and tested with data from respected sources such as the Undiagnosed Disease Program and NHGRI GREGOR Consortium datasets found in dbGaP, RareInsight ensures accuracy and reliability.
RareInsight aids informed decision-making for clinicians and patients alike and fosters collaboration among researchers and clinicians, facilitating seamless sharing and collaboration on reports. By promoting knowledge exchange, RareInsight aims to enrich the collective understanding of rare diseases and the enhancement of healthcare outcomes in an open-source format.
Our goal is to revolutionize rare disease diagnosis and research by leveraging collaboration and innovation, ultimately reshaping the landscape of healthcare.
Early Colorectal Cancer Detection using AI imaging
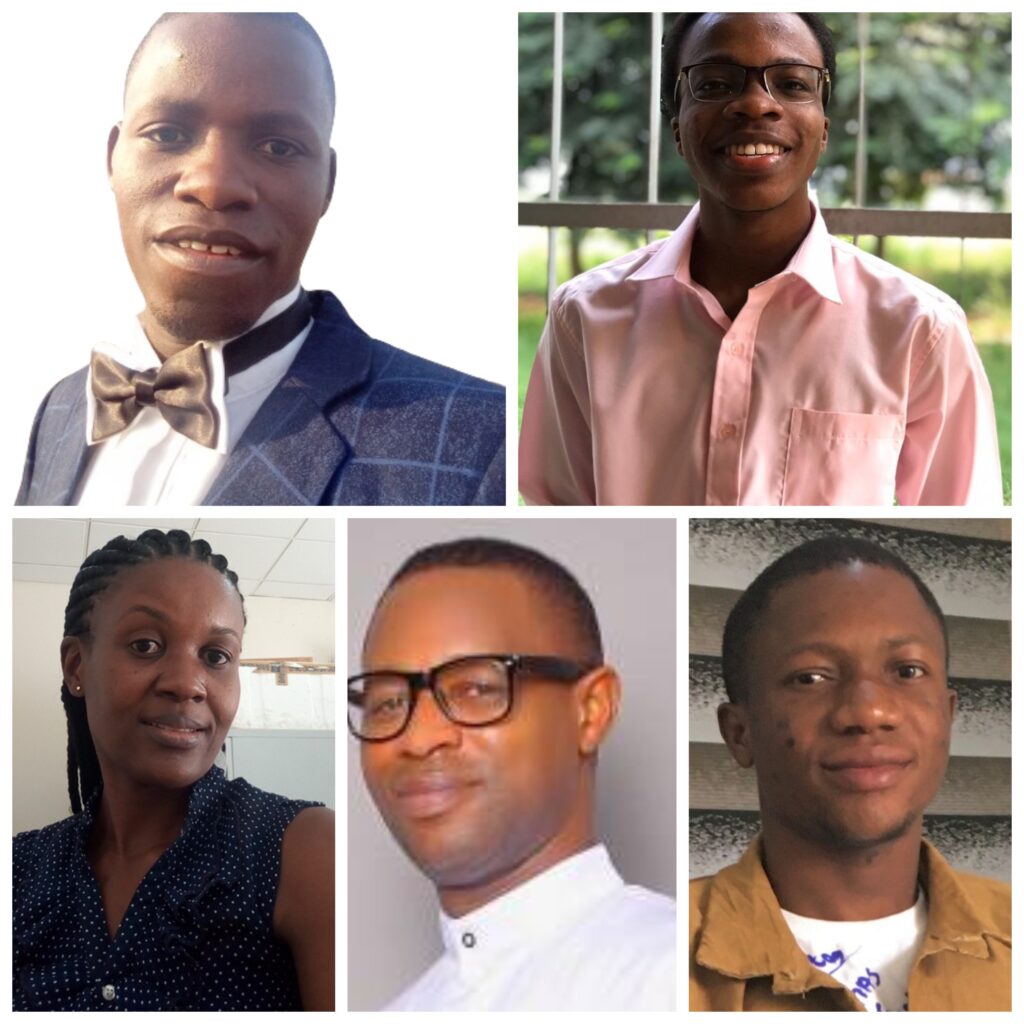
Lawrence Muwonge, Mawunyo Avornyo, Naa Adjeley Frempong, Donald Udah and Daniel Adediran
Colorectal cancer (CRC) remains a significant global health challenge, emphasizing the urgent need for innovative and efficient screening methods. This research introduces an approach to early colorectal cancer detection through the integration of artificial intelligence (AI) and advanced imaging technology. In this study, we leveraged state-of-the-art deep learning algorithms for CRC screening. Our study demonstrates the accuracy and reliability of AI in identifying subtle abnormalities indicative of early-stage colorectal cancer.
This research involves the analysis of a comprehensive dataset comprising diverse histopathology images. The AI model, trained on this dataset, exhibits a high sensitivity and specificity in recognizing early-stage colorectal lesions. This represents a critical stride towards enhancing colorectal cancer screening programs, paving the way for earlier intervention and improved patient outcomes.
These findings not only underscore the transformative potential of AI in healthcare but also reinforce the importance of continued innovation in the quest for early cancer detection. By pushing the boundaries of technology and medical science, we aim to contribute to the ongoing efforts in reducing the global burden of colorectal cancer and improving the quality of life for affected individuals.
Drug Repurposing for the Management of TAU Pathology
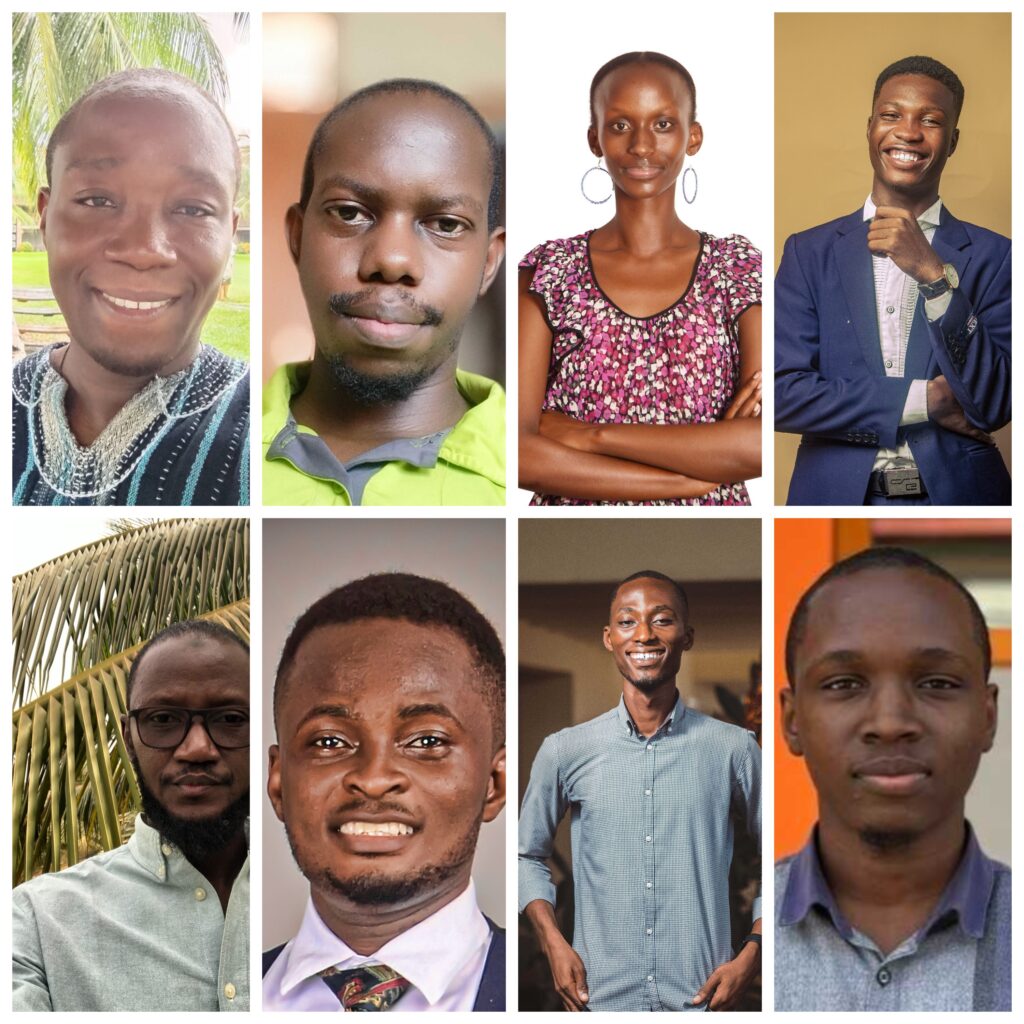
Edward Jenner Tettevi, Mark Kivumbi, Sophia Mogere, Pius Kwasi Sam, Lassana Coulibaly, Opeoluwa Shodipe, Emmanuel Adu Sarpong and David Ojo
The project aims to expedite Alzheimer’s disease (AD) treatment development by repurposing approved drugs. In-silico tools were used to identify potential candidates. Existing drugs, investigational compounds, and drugs in clinical trials were analyzed. Various tools and methods were employed, such as molecular docking, predictive models, scoring algorithms, and molecular dynamics simulations. Molecular docking assesses drug binding to AD-related targets. Scoring algorithms evaluate drug-target binding affinity, predictive models estimate pharmacokinetic properties, and molecular dynamics simulations explore drug-target interactions. By combining these computational approaches with comprehensive drug datasets, the project aims to identify promising candidates for AD. The integration of computational methods in drug repurposing can expedite the discovery and development of effective AD therapies, benefiting patients with this neurodegenerative disease-
In-silico Screening of Natural Compounds as Potential Inhibitors of Aldose Reductase in the Treatment of Type II Diabetes Mellitus
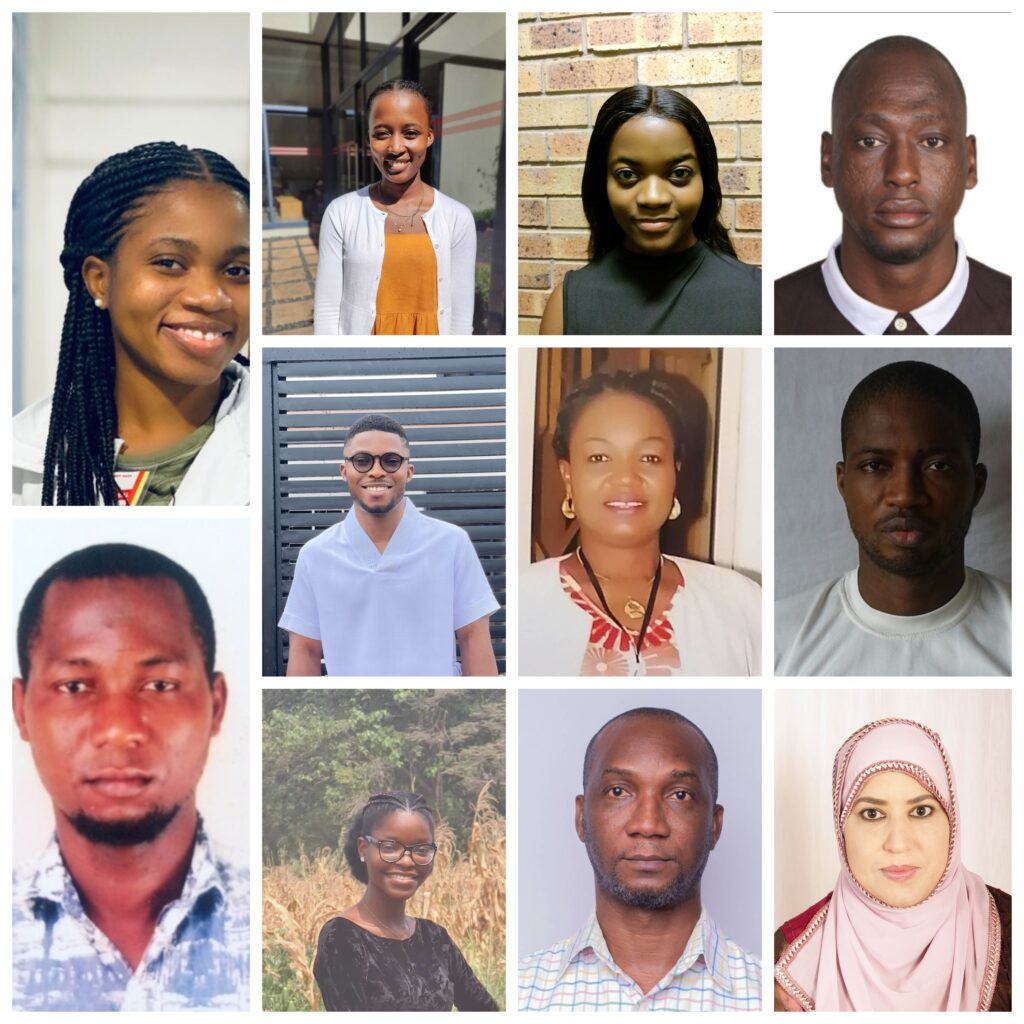
Miriam E.L. Gakpey, Toheeb Jumah, Siyabonga Msipa, Shadrack Arhin Aidoo, Bukola Omonijo, Christabela Palesa Tjale, Florence Mbaoji, Collins A. Onyeto, Mamadou Sangare, Adekilekun Toyyib Adedapo and Hedia Tebourbi
Type 2 Diabetes Mellitus (T2DM) still poses a significant healthcare challenge within the African continent and the world at large. This menace has been primarily attributed to macrovascular and microvascular complications arising from hyperglycemia. While glycemic control remains paramount, adjunctive therapeutic strategies targeting specific pathways implicated in diabetic complications offer promising avenues for improving associated morbidity.
Our research focuses on the aldose reductase (AR) enzyme, a key enzyme in glucose metabolism whose activity and metabolites under high glucose conditions trigger different pathways resulting in diabetic complications. Unfortunately, existing AR-targeting drugs come with limitations such as toxicity, adverse reactions, and a lack of specificity. In this study, we explored the potential of indigenous African compounds, as inhibitors of AR for effective pharmacological intervention.
To achieve this, we employed computational techniques like molecular docking and molecular dynamics simulations where we scrutinized natural compounds sourced from databases for their inhibitory efficacy against AR. The drug-likeness and ADMET (Absorption, Distribution, Metabolism, Excretion, and Toxicity) properties of successfully screened lead compounds were selected. Molecular dynamics stimulations determined the free binding energy of the protein-ligand complexes using molecular mechanics with generalized born and surface area (MMGBSA) solvation.
This study serves as a crucial step toward identifying novel and alternative compounds for inhibiting AR, ultimately paving the way for more effective treatments for type 2 diabetes mellitus and its associated complications.
Unraveling the Genomic Landscape of Anti-Epileptic Drug Resistance: A Bioinformatics approach
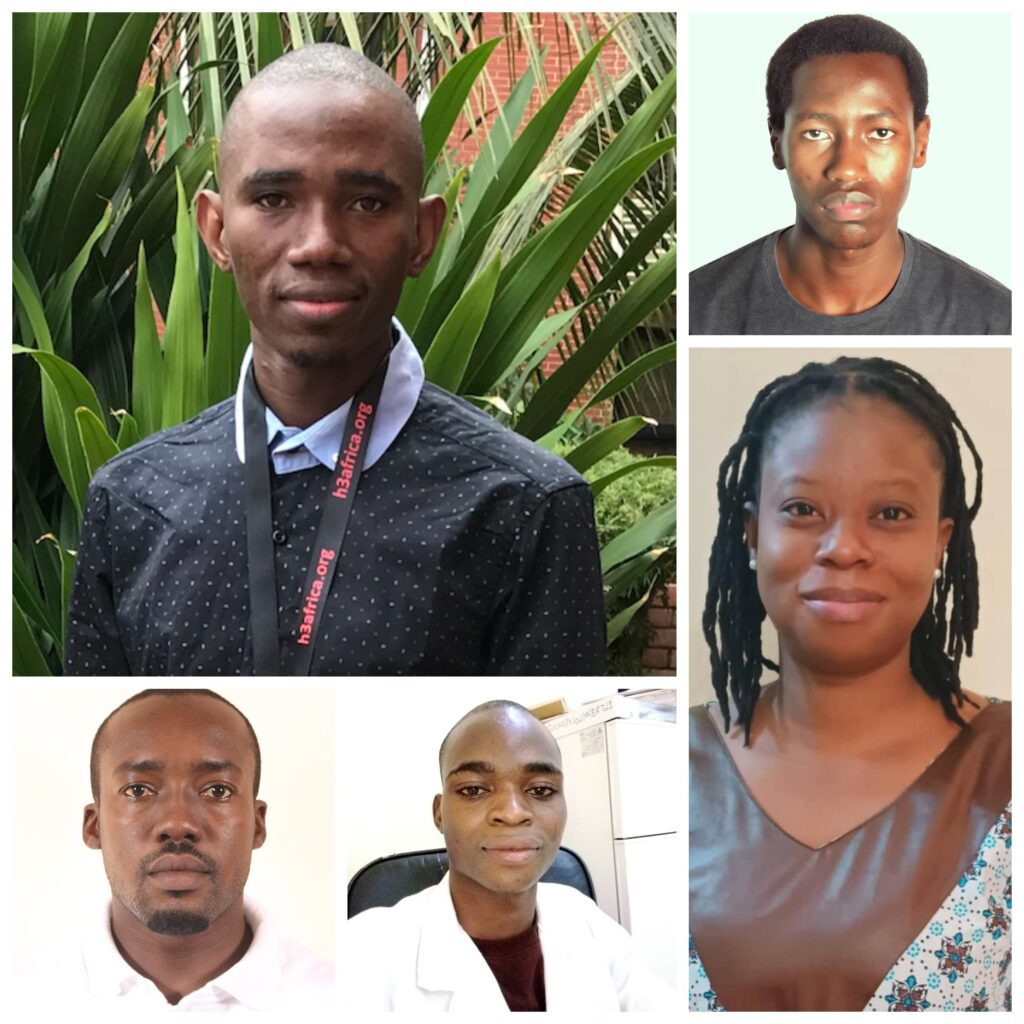
Modibo K. Goita, Alvin Kiprop, Logonia Bernard, Oumarou Soro and Fatoumata G. Fofana
Epilepsy is a chronic neurological disorder characterized by recurring spontaneous seizures. It is one of the most common and most disabling chronic neurological disorders affecting more than seventy million individuals worldwide. More than 20 antiseizure drugs are available for the symptomatic treatment of epileptic seizures, but drug resistance appears in around 30% of patients, leading to unsuccessful treatments. Despite various research efforts, the exact mechanisms underlying drug resistance are not fully understood. Unraveling the genomic landscape of anti-epileptic drug resistance represents a pioneering endeavor in the field of epilepsy treatment. In this study, we employed advanced bioinformatics methods to delve into the intricate genetic makeup of individuals with epilepsy to decipher the underlying mechanisms driving resistance to anti-epileptic drugs (AEDs). By analyzing vast genomic datasets, including bulk RNA sequences, we identified key genetic variants, gene expression patterns, and molecular pathways associated with AED resistance. This comprehensive approach not only enhances our understanding of the complex interplay between genetics and drug response but also holds promise for the development of personalized therapeutic strategies tailored to individuals living with epilepsy.
T-cell Multi-epitope Vaccine Development Against Nipah Virus B Strain
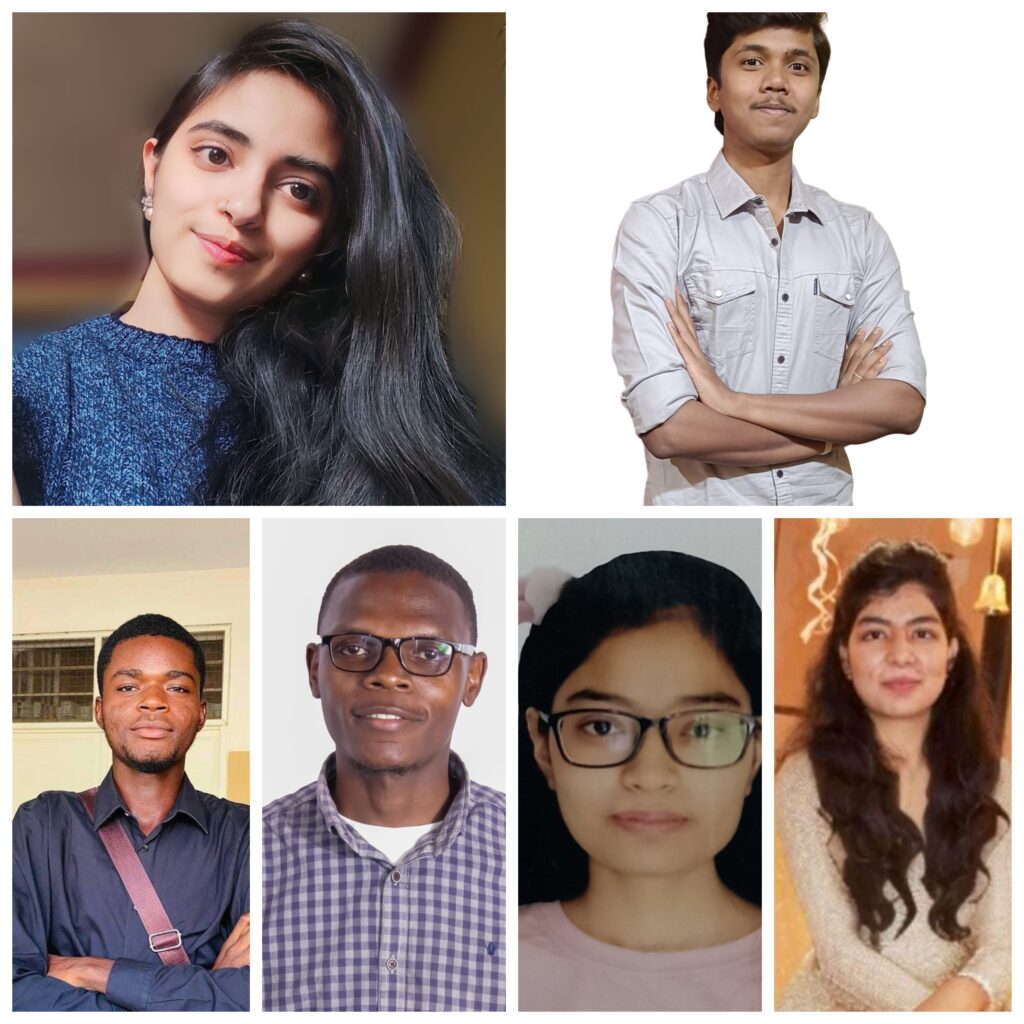
Renuka A. Jojare, Raj Gondane, Arnold Abakah, Samuel Chenge, Prachi Tayade and Shruti Thorle
Nipah Virus (NiV), notably the NiV-B strain, presents a persistent public health concern due to its high mortality rates. Our research adopts a systematic approach, integrating advanced bioinformatics and immunological principles to pinpoint specific T-cell epitopes critical for immune recognition and response against NiV-B. With a focus on key structural glycoproteins and nucleoproteins, we aim to delineate immunogenic regions that can serve as targets for vaccine development.
Utilizing computational modeling and rigorous analytical techniques, we sought to characterize epitopes with high binding affinity to major histocompatibility complex (MHC) molecules, ensuring efficient antigen presentation and T-cell activation. By harnessing insights from immunoinformatics and epitope prediction algorithms, we designed a tailored multi-epitope vaccine capable of eliciting robust and durable immune responses.
Our methodology prioritized rational vaccine design, leveraging empirical data and computational tools to expedite the discovery process while minimizing resource expenditure. Through in-depth characterization of NiV-B epitopes and their interactions with host immune receptors, our study aims to enhance our understanding of protective immune mechanisms against NiV infection.
Ultimately, our research contributes to the development of evidence-based vaccination strategies against NiV-B, offering a pragmatic and scientifically grounded approach to mitigating the threat posed by this emerging pathogen.
Drug Candidate Development against Non-alcoholic Steatohepatitis (NASH)
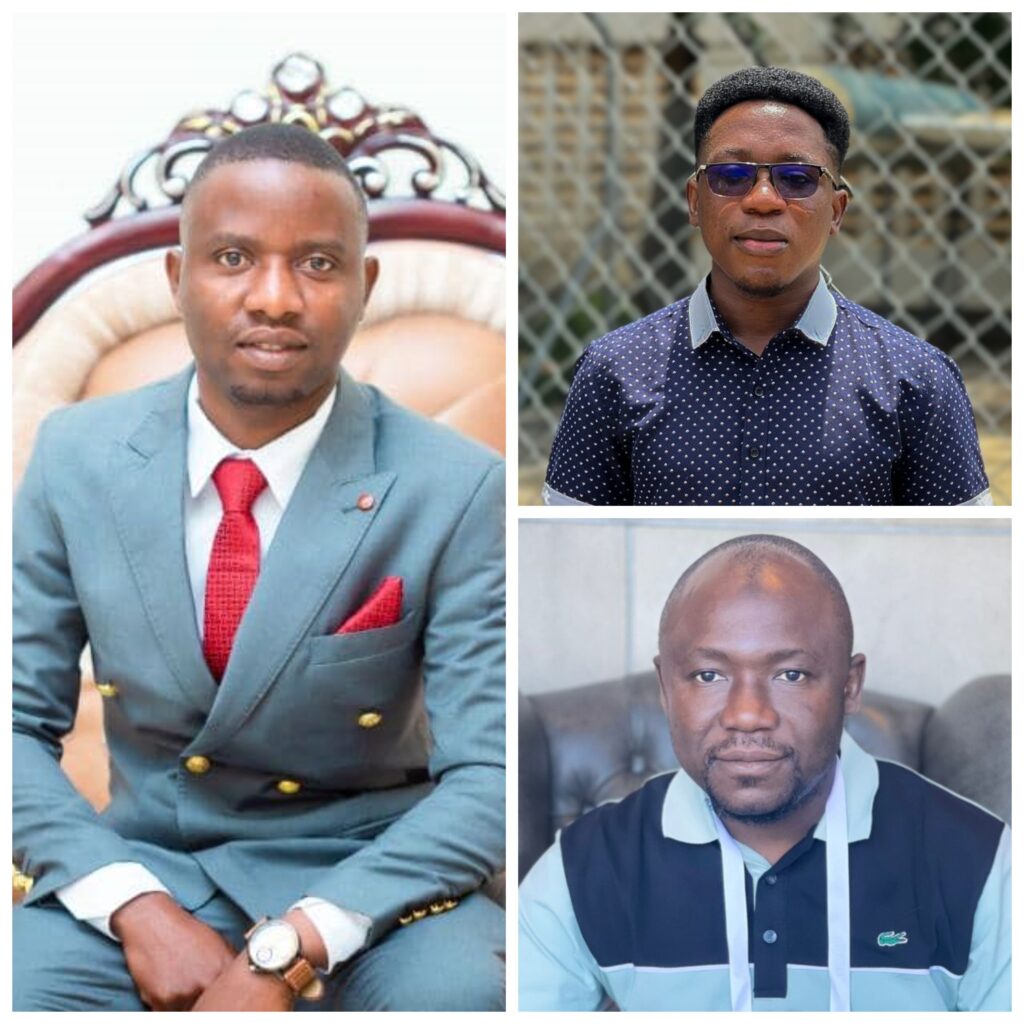
Chimenya Ntweya, Desmond Osarfo Amoah and Diabate Oudou
Nonalcoholic fatty liver disease (NAFLD) is characterized by the abnormal accumulation of fat in the liver (hepatic steatosis) in the absence of other causes of secondary liver fat accumulation. NAFLD encompasses both nonalcoholic fatty liver (NAFL) and nonalcoholic steatohepatitis (NASH), which is diagnosed when there is evidence of inflammatory activity and hepatocyte injury in steatotic liver tissue. NASH represents the most severe form of NAFLD, wherein excessive fat deposits accumulate in the liver. Unlike NAFL, NASH carries the highest risk of progressing to cirrhosis, liver failure, and liver cancer. The global
prevalence of NASH exceeds 115 million adults, a number projected to rise by 2030, imposing substantial burdens on individuals, families, and economies. Currently, there are no approved medications specifically for treating NASH. Therefore, this project aims to identify potential therapies for NASH using computational methods. In this study, we utilized a series
of in-silico techniques such as homology modeling, ligand-based pharmacophore modeling, molecular docking, and molecular dynamic simulations to identify potential drug candidates for treating NASH. Our research makes a major contribution to the ongoing effort to develop drugs for NASH.
Methylomic Variations Distinguishing Temporal Lobe Epilepsy Subtypes: Focus on Hippocampal Sclerosis
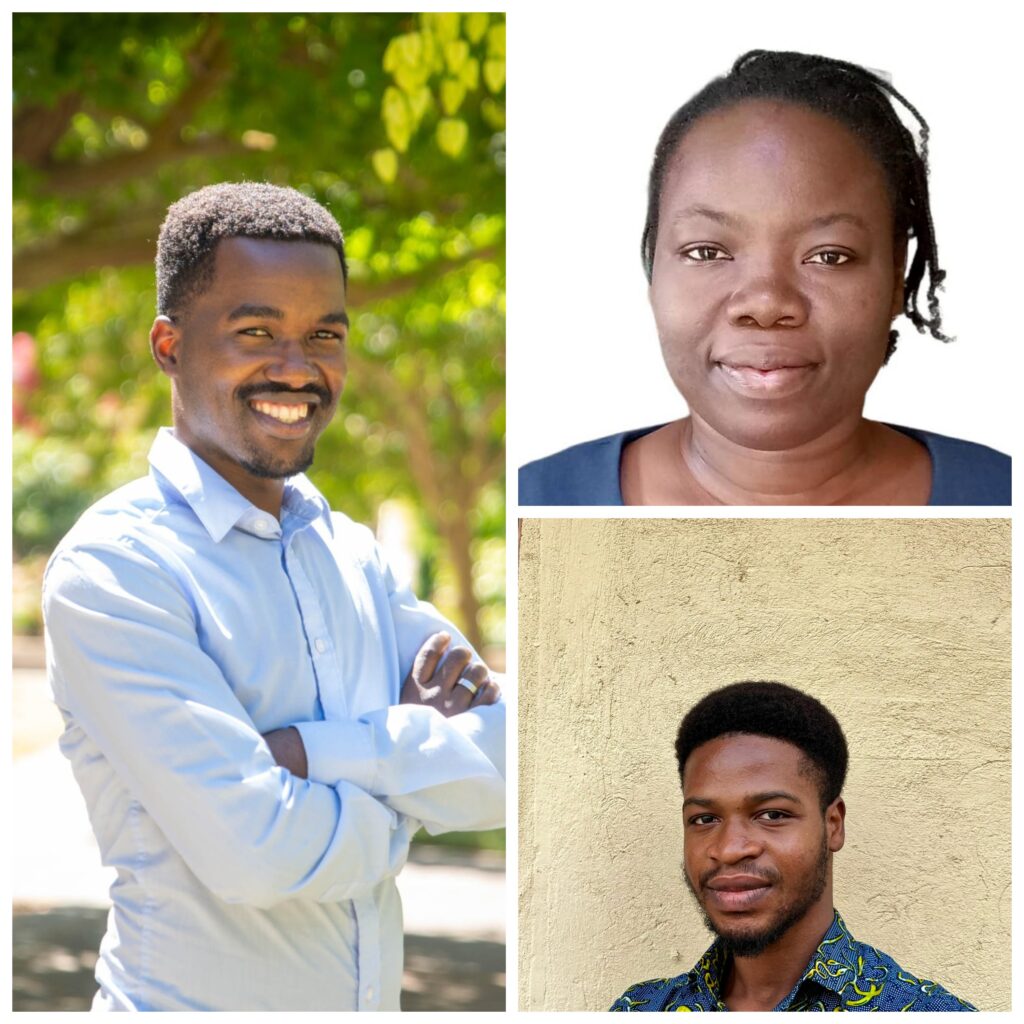
George Eusebio Kuodza, Ifeoluwa Hephzibah Ojelabi and Jonathan Kalami
Epilepsy, is one of the most prevalent neurological conditions, which presents as a complex disorder, characterized by recurrent seizures with excessive and abnormal neuronal discharges, it has been attributed to both genetic and environmental factors. Gene regulation of epigenetics basically includes DNA methylation, histone modification and RNA methylation, so as to change the genetic information of organisms without altering the sequence of DNA nucleotides. Our focus in this study is on the DNA methylation so as to give plausible answers for frequent characteristics of complicated disorders. We identified the methylated regions in patients with epilepsy using Bisulfite mapping and methylation calling, comparing differences in methylation among those that have temporal lobe epilepsy with hippocampal sclerosis and those with temporal lobe epilepsy without hippocampal sclerosis. Our study’s result will give insight into the different effects of DNA methylation in epilepsy and possibly the molecular mechanisms underlying the complications. With this understanding, personalized treatments may be developed.
Predictive Models for Malaria Susceptibility in Diverse African Populations based on Sex and Age Groups
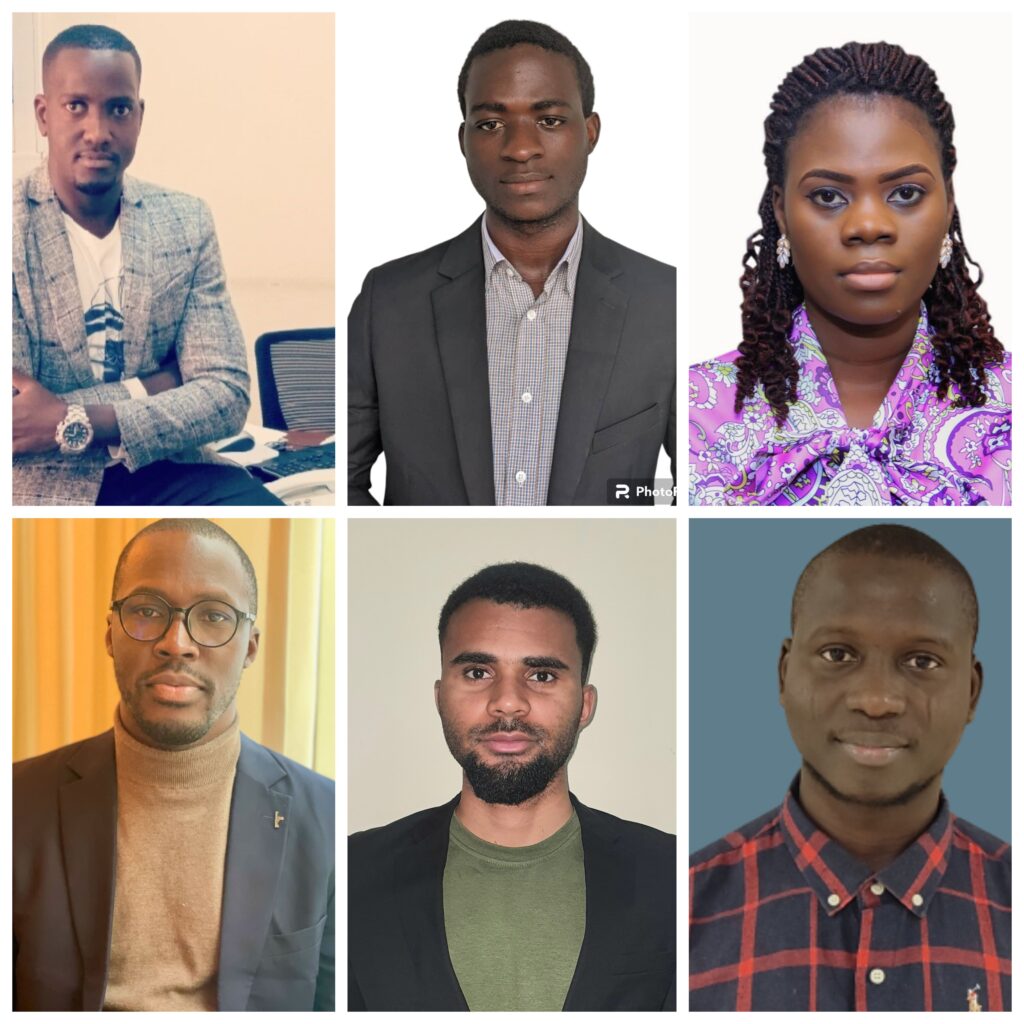
Mamadou D. Coulibaly, Ndong H. Ndang, Oyepeju D. Atarase, Fousseyni Kane, Adham Hallal and Abdulwasiu Tiamiyu
Malaria susceptibility is the predisposition to be infected by malaria parasites. Factors influencing susceptibility to malaria parasites could be genetic or generic such as age, sex composition, social and economic status as well as mobility of the population. These factors create a difference in susceptibility rates among populations. Unfortunately, certain populations become more susceptible to malaria infection than others. It is therefore key to understanding in detail populations more susceptible to infections first to inform policy and then implement cost-effective interventions with priority given to populations with higher susceptibility to malaria. In this project, we analyzed and modeled genomic data consisting of biological susceptibility factors such as age and sex using machine learning to predict malaria susceptibility in diverse African populations.
Investigating the Molecular Interplay between HIV1 and Influenza/SARS-CoV-2 Infection through Integrative Gene Expression Analysis
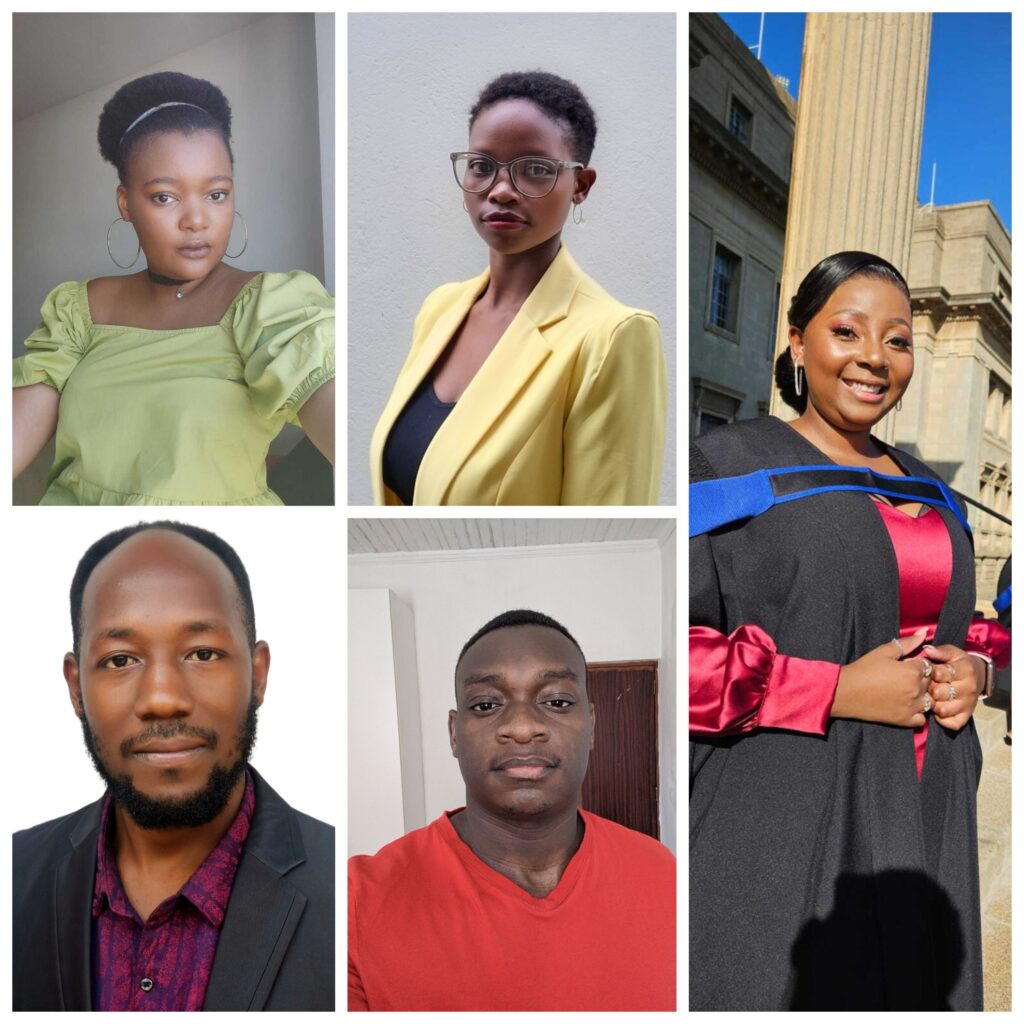
Sibongiseni Msipa, Marion N. Nyamari, Palesa Lesole, Fredrick E. Kakembo and Precious Kunyenje
We aim to address the need for innovative strategies in managing the intricate relationship between SARS-CoV-2/Influenza and HIV infection. Using integrative gene expression analysis, we seek to propel targeted interventions. Our core objective is to construct a robust framework empowered by bioinformatics tools and machine learning algorithms. This framework is designed to unravel the complex molecular mechanisms driving co-infection pathogenesis. Through transcriptomics and network analysis, we aim to identify novel therapeutic targets, and refining personalized treatment for affected individuals.
Our methodology involved RNA-seq analysis and gene expression profiling obtained from SARS-CoV-2 and HIV infected patients with the goal of implementing a Nextflow DSL2 bioinformatics pipeline. This analysis aims to delineate regulatory pathways and dysregulated genes associated with disease progression, shedding light on both commonalities and distinctions in molecular signatures underlying co-infection. By meticulously integrating results from individual infection analyses, we aim to unveil potential interactions and synergistic effects, thus providing invaluable insights into the molecular mechanisms steering co-pathogenesis and ultimately enhancing clinical outcomes for affected individuals.We firmly believe that our integrative gene expression analysis framework harbors transformative potential in elucidating the molecular underpinnings of SARS-CoV-2 and HIV infection, thereby paving the way for more effective interventions.
Comparative Analysis of DESEQ2 and edgeR Differential Gene Expression Methods in Triple-Negative Breast Cancer
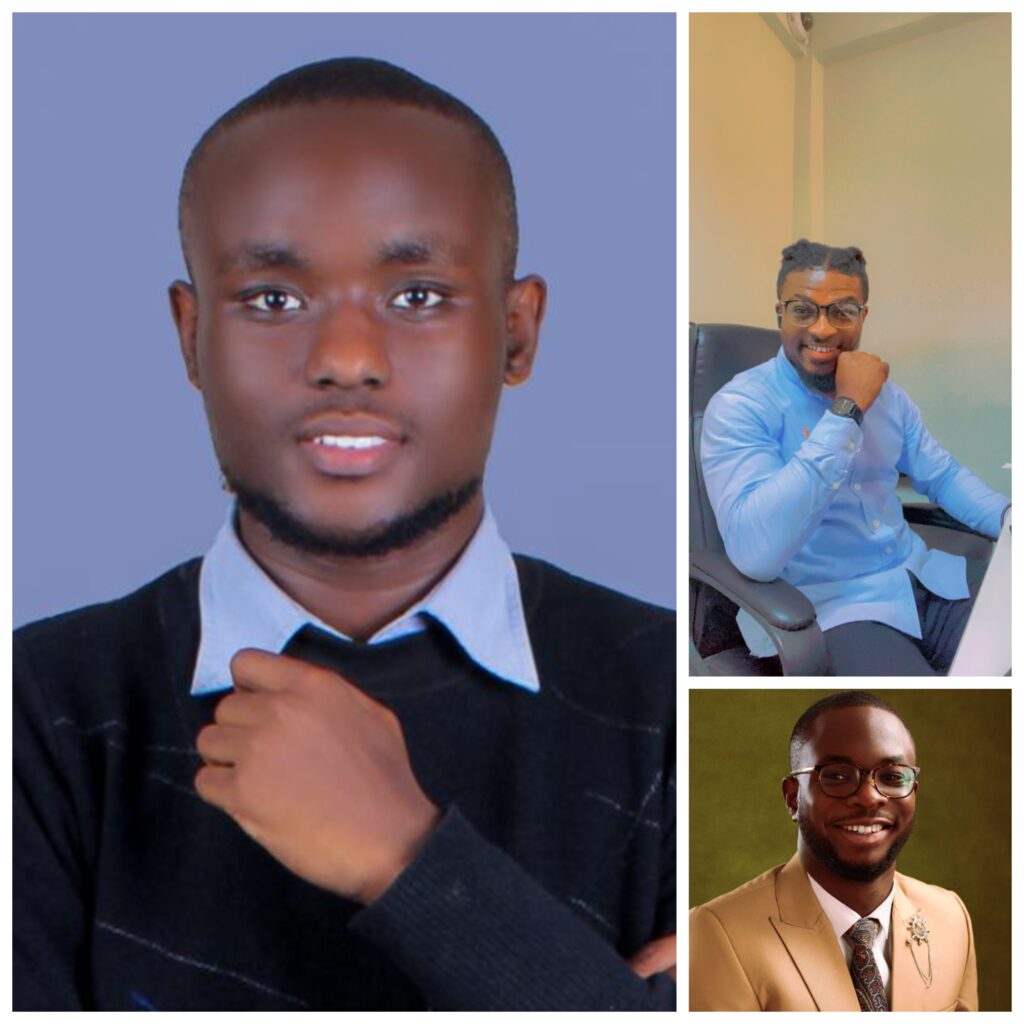
Martin Njau Kamau, Enock Kofi Amaoko and Felix Oluwasegun Ishabiyi
Breast cancer is a complex disease encompassing various subtypes, including Triple-Negative Breast Cancer (TNBC), characterized by the absence of estrogen, progesterone, and HER2 receptors. Our project focuses on evaluating two vital differential gene expression analysis tools in cancer research, DESeq2 and edgeR, which play key roles in identifying genes that are significantly dysregulated in TNBC.
Our primary goal is to conduct a rigorous comparative analysis between DESeq2 and edgeR in TNBC using RNA-seq data. We aim to assess their effectiveness in pinpointing dysregulated genes specific to TNBC, comparing their sensitivity, specificity, and correlation coefficients in detecting differentially expressed genes, and evaluating the statistical significance and robustness of their results.
This study holds immense potential for contributing to TNBC research, including the identification of novel therapeutic targets and biomarkers. These findings can directly translate into clinical practice, leading to improved diagnosis and treatment strategies for TNBC patients.
Machine Learning Models, Molecular Docking and Simulation for the Prediction of Novel Small Molecules as Potential TGR5/GLP-1 Agonists in Type II Diabetes Treatment
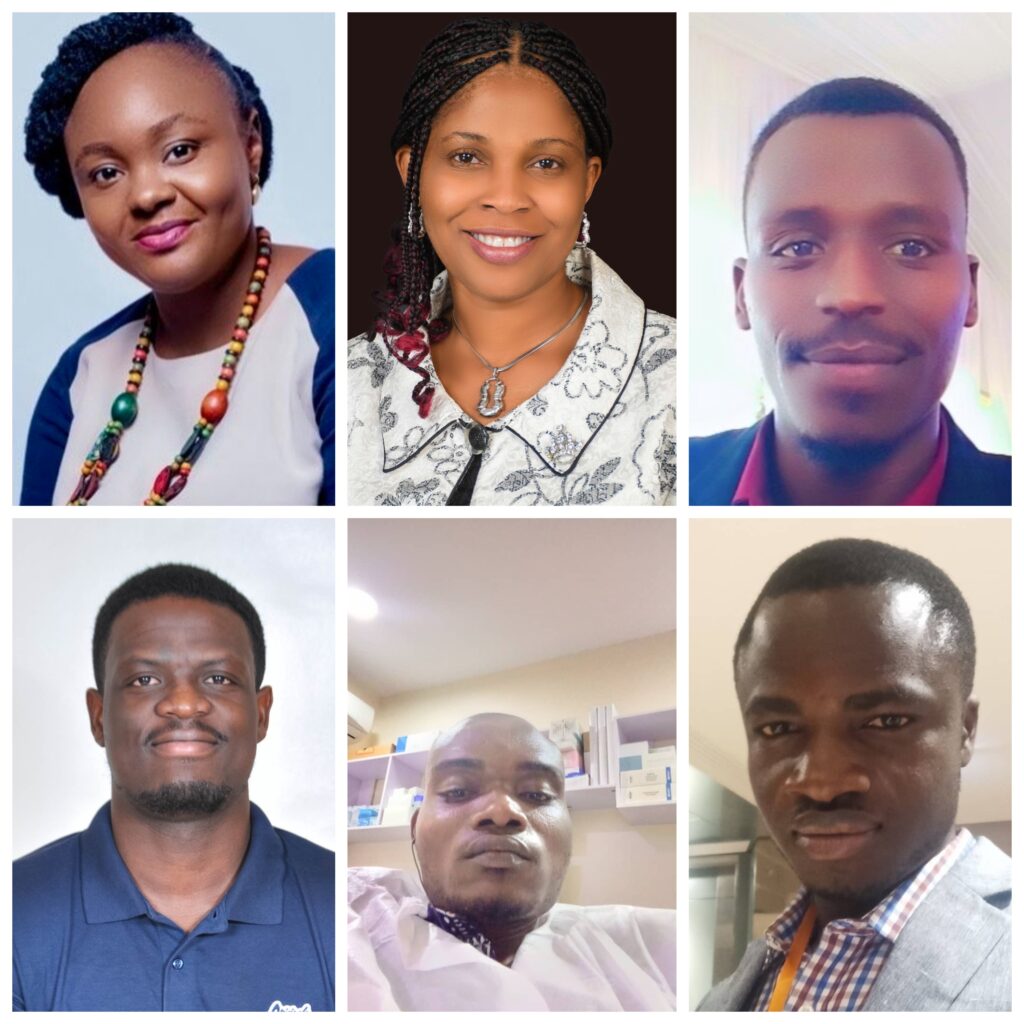
Ojochenemi A. Enejoh, Chinelo Henrietta Okonkwo, Moses Ainembabazi, Hector Nortey, Oluwasegun Adesina Babaleye and David Olatunji
TGR5 agonists have emerged as a promising class of therapeutic agents for the treatment of type 2 diabetes mellitus. Machine learning algorithms combined with molecular docking and simulation techniques provide valuable insights into the interactions between small molecules and TGR5 receptors. This approach not only helps in predicting the potential efficacy of a molecule, but also aids in understanding the molecular mechanisms underlying TGR5 activation.
In this study, to identify novel TGR5 agonists, we employed a machine learning-based modeling approach which involved the development and validation of machine learning-based models to predict the binding affinity of small molecules to TGR5, followed by molecular docking and simulation techniques. This allowed for the virtual screening of a large compound library to identify potential small molecule candidates that can bind to and activate the TGR5 receptor. Moreover, TGR5 agonists have been shown to stimulate GLP-1 secretion, which can lead to improved glycemic control through glucose-dependent insulin secretion. These advancements in the field of diabetes treatment have the potential to significantly improve diabetes management and treatment outcomes.
Longitudinal Metagenome Analysis for Personalized Exposure
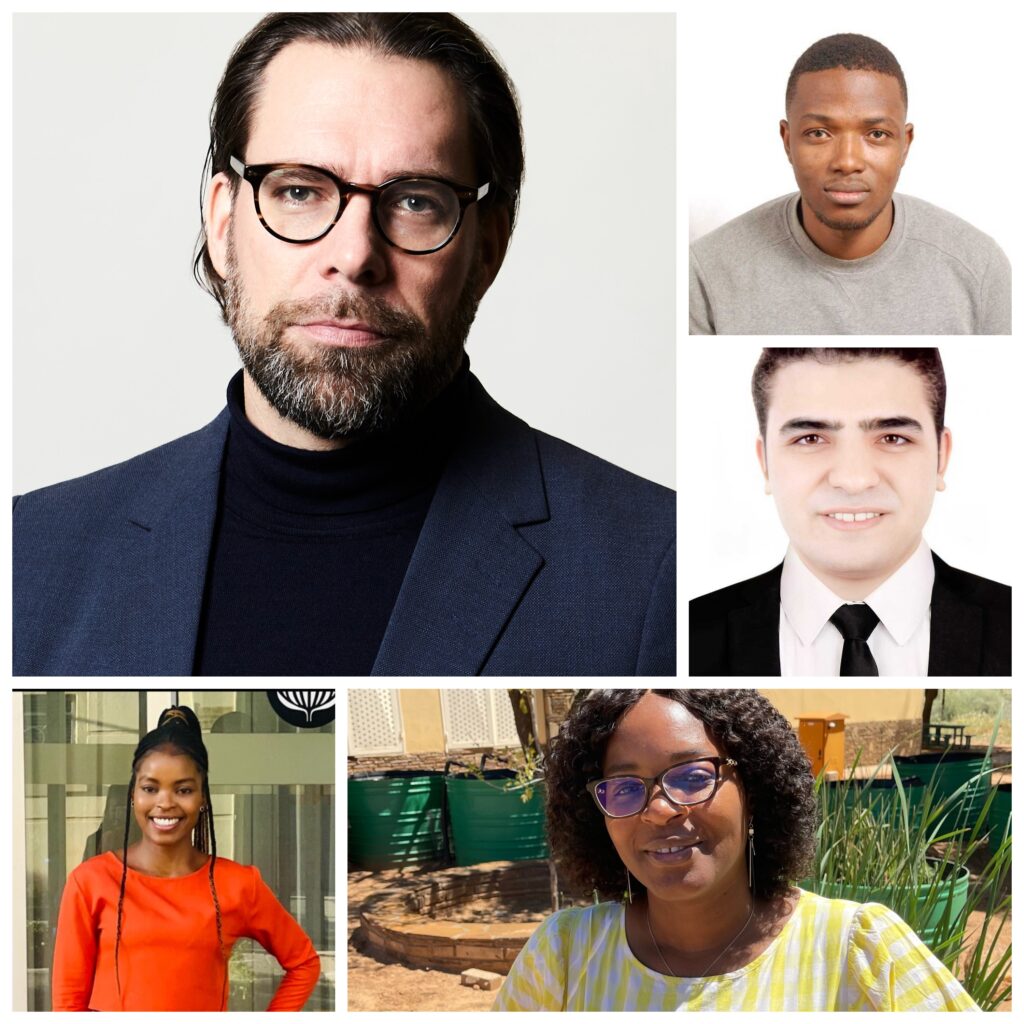
Ville Pimenoff, Albert Rock A. Gangbadja, Mohamed S. AboHoussein, Nonsikelelo Precious Hlongwa and Fadzai Mbiri
Longitudinal aerosol metagenomic profiling will eventually change our understanding on biotic exposures. That is, with wearable devices we can collect longitudinal cumulative aerosol exposure samples from our daily environment. It may even be possible to accurately predict changes in an individual’s health using personalized aerosol metagenomic profiling.
In this project, we assessed the reproducibility of fine-scale metagenomic profiling of microbes (ie. bacteria, virus and fungi) from personalized longitudinal aerosol samples. In particular, we evaluated the diversity and distribution of likely pathogenic microbes present in the personal aerosol profiles. For the project, we have six months bi-weekly personal-aerosol sampled metagenome data available from fifteen individuals. From these previously published aerosol metagenomes, we assessed the fine-scale longitudinal biotic profiles. Our results indicate that the microbial species detected from the personal aerosol sampled metagenomes are highly reproducible and enable longitudinal profiling of likely pathogenic exposures at individual level.