Omics codeathon is an established event where life scientists work on research projects. It is held twice a year and led by Olaitan I. Awe (Ph.D.), the current training officer for the African Society for Bioinformatics and Computational Biology (ASBCB).
The October 2023 codeathon was virtual.
The primary aim of the omics codeathon is to use omic sequences and bioinformatics to advance the understanding of the biology of model organisms and pathogens to ultimately improve human health. Our research projects typically use human, cellular, cancer and pathogen genomics to investigate the molecular mechanisms of mendelian disorders and complex traits. By using different omics approaches, we are interested in trying to understand how diseases work at the molecular level.
Codeathon participants and applicants come from across the globe, including South Africa, Kenya, Nigeria, Libya, Tunisia, Algeria, Zimbabwe, Morocco, Egypt, Senegal, Mali, Ghana, Brazil, Uganda, Poland, Tanzania, Mozambique, Bangladesh, Botswana, Burkina Faso, Gambia, Benin Republic, Ethiopia, South Korea, Saudi Arabia, Sudan, Malawi, Zambia, Cameroon, Conakry-Guinea, Dubai / United Arab Emirates, India, Taiwan, China, Sweden, Finland, Germany, France, Ireland, Spain, Australia, Mexico, United States, United Kingdom and Canada.
The October 2023 codeathon projects were in these categories; Bulk Transcriptomics, Pathogen Genomics, Metagenomics, Human Genomic Variation, Pipeline Development, Biomarker Discovery, Cheminformatics, Clinical Applications, Drug and Vaccine Design, Antimicrobial Resistance, Population Genomics, Genome Wide Association Studies, Polygenic Risk Scores, Mendelian Randomisation, Oncology, Structural Bioinformatics, Plant Genomics, Single Cell Transcriptomics and Machine Learning.
Omics Codeathon Supporters:
- National Institutes of Health, Office of Data Science Strategy (NIH ODSS)
- University of the Western Cape
Projects
Title
Team
Project Description
The lack of reproducible science in proteomics analyses – a bioinformatics perspective
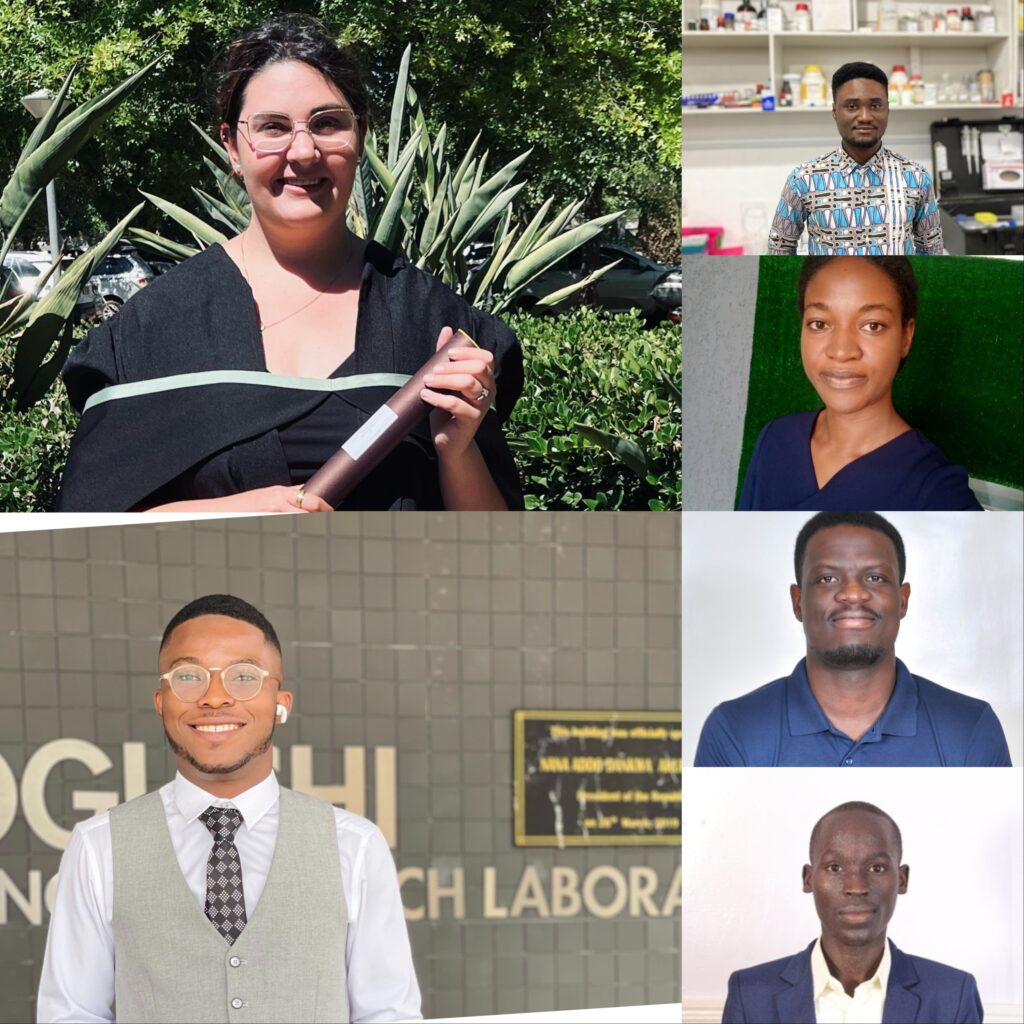
Kimberly Christine Coetzer, Shadrack Arhin Aidoo, Nana Adomako Ansah, Itunu Ajiboye, Hector Nortey and Opio Isaac Okello
In proteomics research, reproducibility poses a significant challenge. The complexity and variability of proteomic data, coupled with the lack of standardized protocols and analysis pipelines, contribute to this issue. In this study, we did a review which aimed to identify the key obstacles and causes behind the lack of reproducibility in proteomics analysis from a bioinformatics perspective, as well as potential solutions. We compiled a list of articles from various sources to gain a comprehensive understanding of the field’s current state and gaps in standardized protocols. The literature review found numerous studies focusing on various aspects of proteomics data analysis, such as data preprocessing, statistical analysis, and result interpretation, highlighting the absence of consensus and standardized approaches. Thus, further exploration is necessary to establish a unified framework that enhances the reproducibility of proteomics data analysis. Our review introduces an approach to assess tools on a reproducibility scale, evaluating their documentation, version control, and testing practices. This scoring system can assist developers in improving their tools and aid researchers and users in selecting reliable and reproducible proteomics data analysis tools.
Attributing the Source of Salmonella Enterica: A Machine Learning Approach to Understanding Pathogen Surveillance in Africa
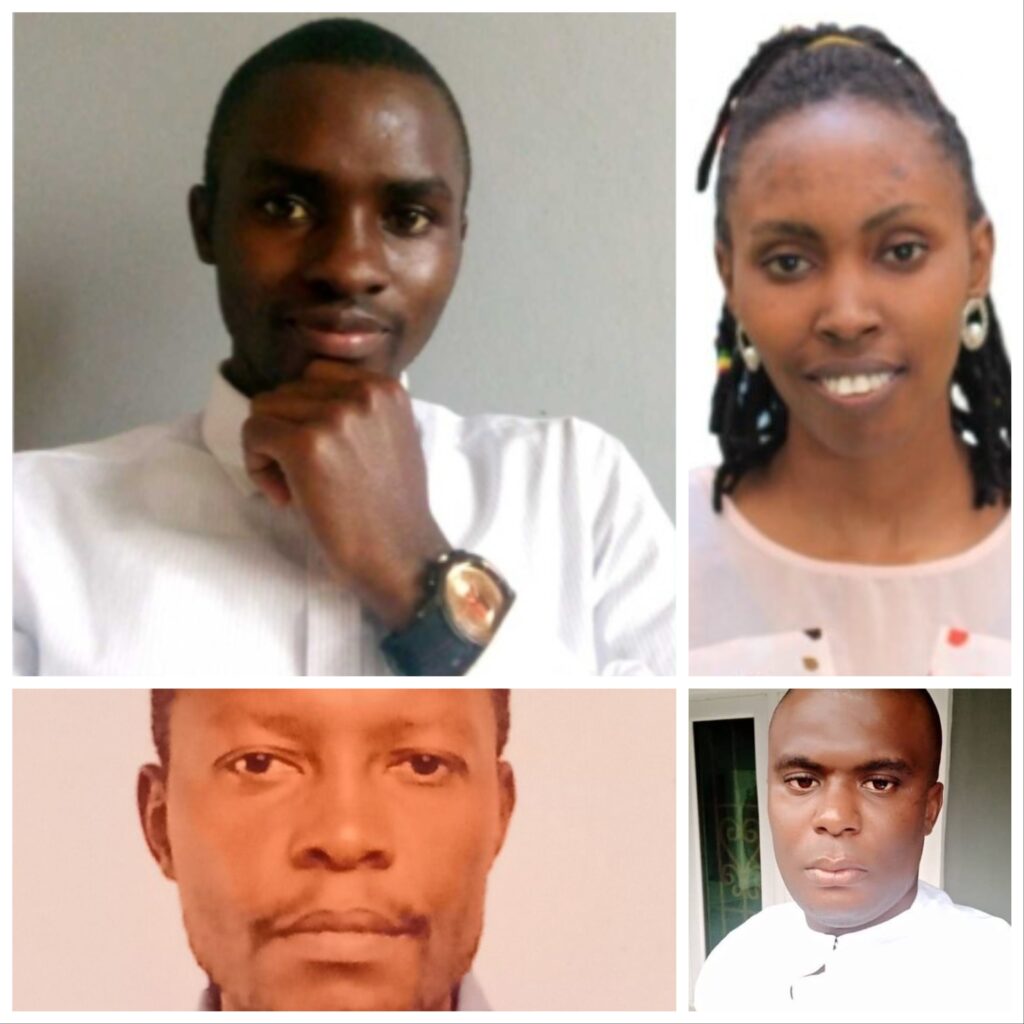
Bonface Onyango, Brenda M. Kamau, Oscar Nyangiri and Asime Oba
Salmonella enterica is a food-born pathogen that can be transmitted from animals to humans and is responsible for causing a significant burden of foodborne illnesses worldwide. Salmonella is spread through the fecal-oral route and can be transmitted through various means, including the ingestion of fecal-contaminated food and water, direct contact with infected animals, and, in rare instances, through person-to-person transmission. These illnesses can manifest as gastroenteritis, fever, abdominal cramps, and, in severe cases, they may lead to hospitalization and fatalities. Developed countries have made strides in mitigating Salmonella infections through advancements in food safety practices, public health infrastructure, and modern technologies. However, Salmonella enterica remains a significant public health burden in low and mid-income countries. To address the challenges faced in low-resource settings, we employed machine learning techniques to attribute the potential reservoir sources of Salmonella enterica by utilizing publicly available pathogen surveillance datasets from selected African countries. The pathogen sources are categorized into bovine, poultry, human, and swine. This approach would facilitate targeted interventions and tracking pathogen transmission routes leading to more efficient prevention and control strategies in African settings.
Investigating the Molecular Connections between Natural Phytochemicals and Type 2 Diabetes
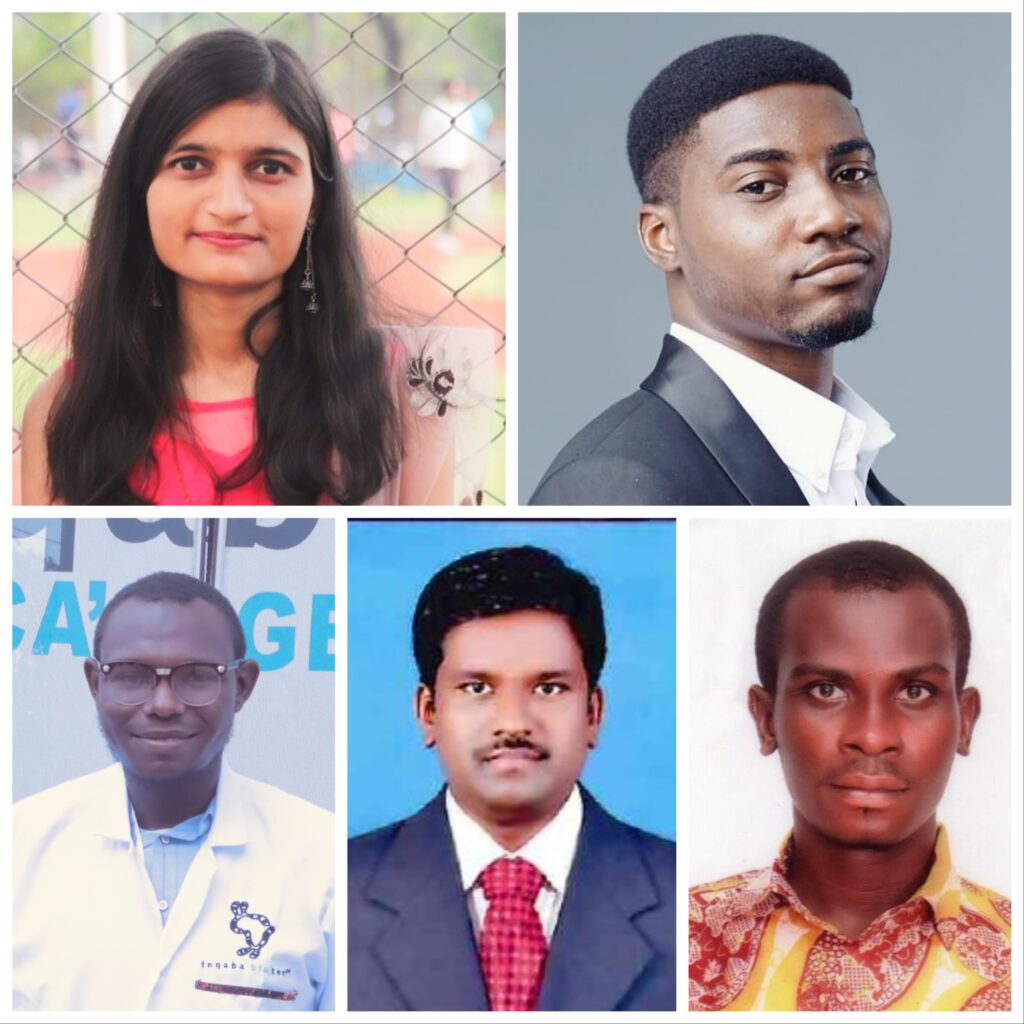
Shivani Pawar, Nigel Dolling, Musa Muhammad Shamsudeen, Dr. Magudeeswaran Sivanandam and Raphael Abban
This project aims to uncover deeper insights into the molecular associations between phytochemicals and receptors relevant to Type 2 diabetes. Our research could provide a better understanding of how natural phytochemicals modulate pathways linked to T2D. The computational methodologies employed could expedite the identification of potential natural compounds for innovative antidiabetic medications. To achieve these objectives, we chose a selection of 10 plant species widely distributed across Asia and Africa, acknowledged for their therapeutic potential against diabetes, named Momordica charantia L., Trigonella foenum-graecum L, Moringa oleifera Lam, etc. These plants have been meticulously studied, and approximately 200 phytochemicals have been extracted from them.
In this project, we performed virtual screening, to discern and isolate drug-like compounds and phytochemicals that exhibit the desired characteristics. Subsequently, to scrutinize the interactions between these identified phytochemicals and the potentially influential receptor proteins associated with T2D, our project employed the technique of molecular docking and molecular dynamics (MD) simulations. This enables a closer examination of whether these phytochemicals induce activation or inhibition within the receptor proteins. This comprehensive computational study investigated the connections existing between phytochemicals and receptors of Type 2 diabetes. By revealing these connections, the project offers promising avenues for advancing therapeutic strategies in diabetes management.
Enhancing Early Breast Cancer Intervention with AI Imaging
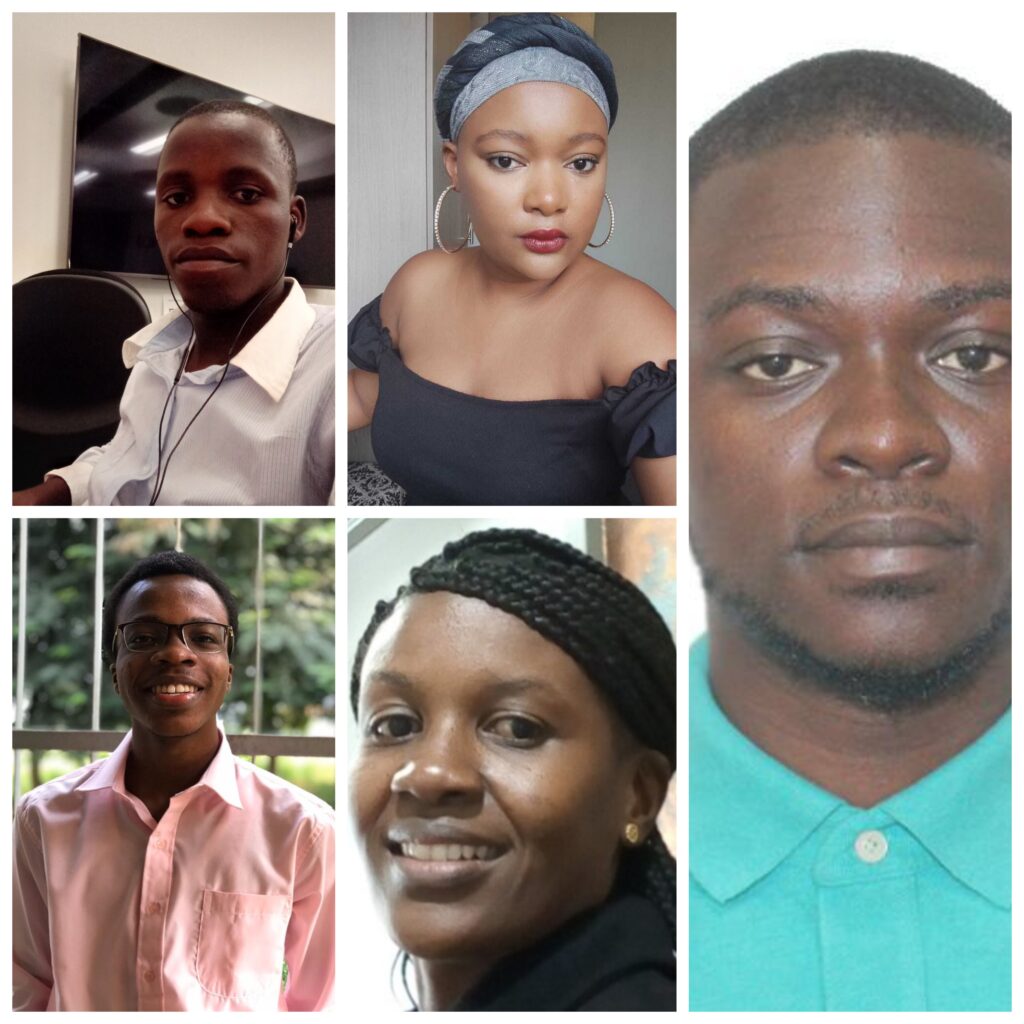
Lawrence Muwonge, Sibongiseni Msipa, Mawunyo Avornyo, Naa Adjeley Frempong and Emmanuel Osei-Frempong
Addressing the escalating global breast cancer challenge and the limitations of current detection methods, our project harnesses AI imaging’s potential to enable timely interventions. Our central aim is to establish an AI-driven framework that heightens breast cancer detection accuracy, offers vital radiologist support, and tailors treatments to individual needs. By integrating cutting-edge techniques like deep learning and convolutional neural networks (CNNs), our mission is committed to enhancing precision, reducing false positives, and fostering data-driven decision-making. Our ultimate vision paints a future where the fusion of technology and medical expertise harmoniously reshapes breast cancer screening and patient care, ushering in a new era of proactive, personalized strategies.
In this study, we proposed and implemented an approach for early breast cancer intervention through AI imaging and it involves a two-phase process: initial image classification, utilizing a CNN model to categorize input images as benign or malignant; and subsequent semantic segmentation, utilizing another CNN to accurately pinpoint the location of masses or breast calcifications within the input image. The classification CNN will be trained on a dataset of labeled breast cancer images categorized as benign or malignant. We firmly believe that our AI-powered framework holds the transformative potential to revolutionize breast cancer detection and treatment paradigms.
Detecting the Novel Markers of Autism using Machine Learning and scRNA-seq
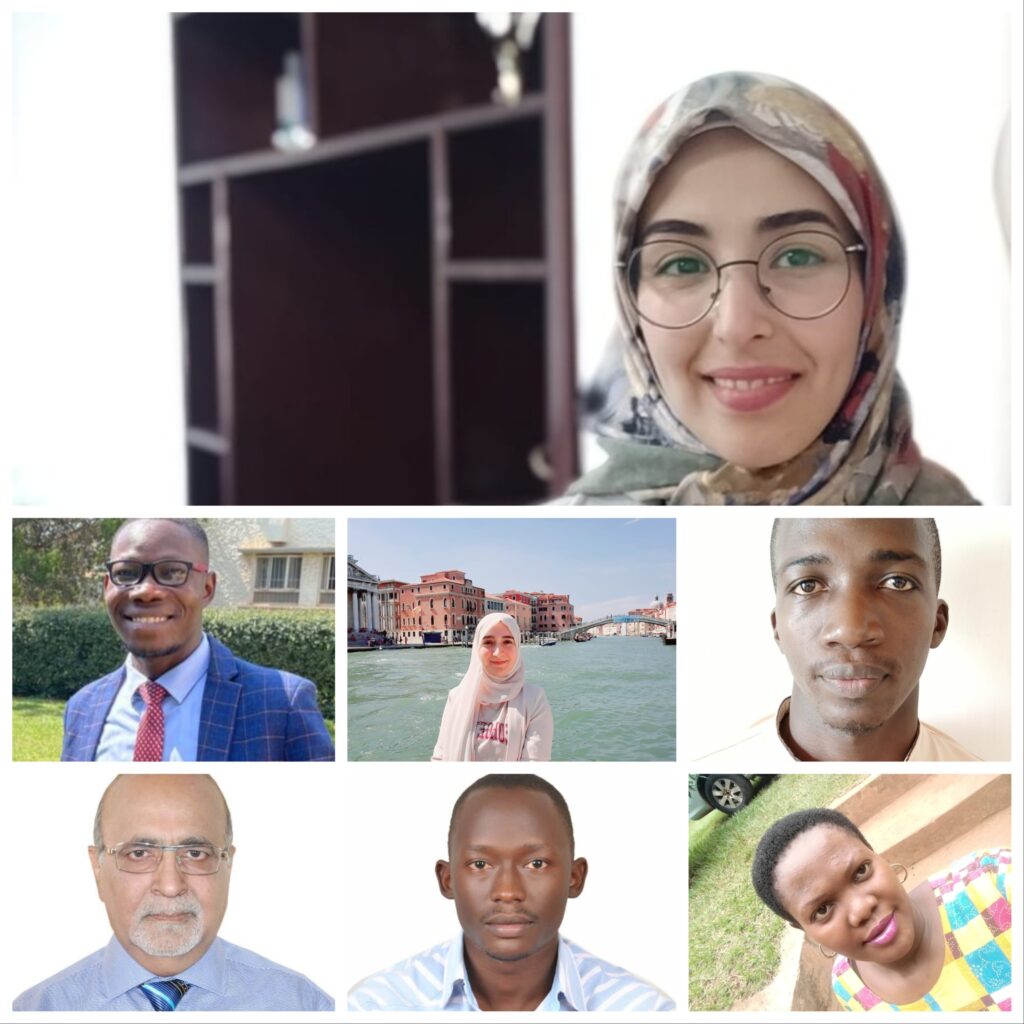
Fatima Zahra Annassiri, Ronald Ogoola, Nouhaila En najih, Steve Bicko, Dr. Mohammed Raza, Herbert Agasi and Catherine Nabbumba
This project aims to develop an automated pipeline to identify potential biomarkers linked to Autism Spectrum Disorder (ASD). By utilizing machine learning techniques and single-cell RNA sequencing (scRNA-seq) data, our project focuses on analyzing samples from 14 ASD patients and 6 controls. These samples, collected from the Prefrontal Cortex (PFC) and Anterior Cingulate Cortex (ACC) brain tissues, hold key insights into ASD’s genetic basis. Our project’s methodology harnesses machine learning algorithms to uncover unique gene expression patterns that could serve as valuable biomarkers for ASD diagnosis and treatment. Our ultimate goal is to enhance our understanding of ASD and provide better avenues for intervention and care. Data for our project was sourced from the reputable NCBI Sequence Read Archive, thereby ensuring robust and reliable analysis.
Construction of Multiple Epitope Vaccine Against H3N8 Strain of Avian Influenza: A Bioinformatics Approach
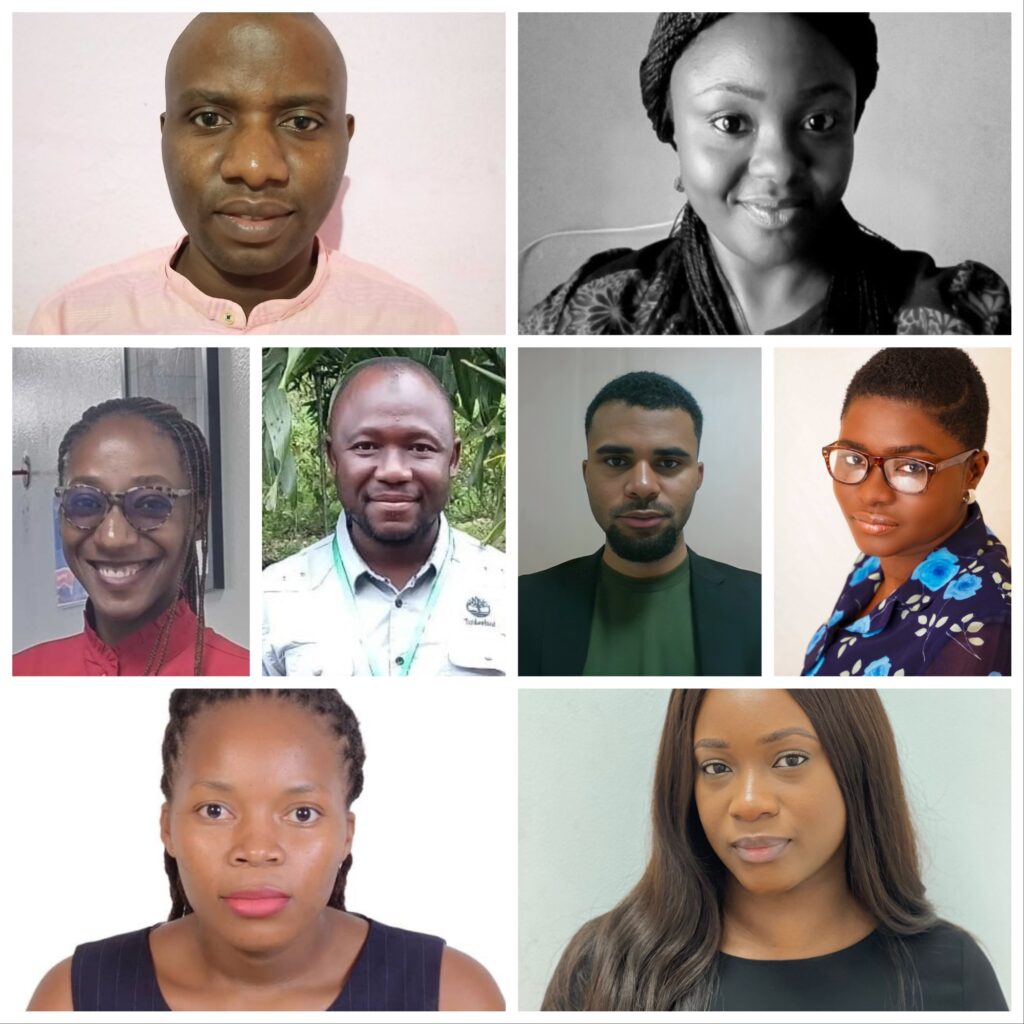
Chimenya Ntweya, Ojochenemi A. Enejoh, Deborah Ayando, Oudou Diabate, Adham Hallal, Margaret Oyekunle, Anjellina Rukundo and Eniola Onabowale
Avian influenza has been classified as a variant of concern by the World Health Organisation due to the spike protein’s numerous mutations which have been found to evade the effects of antibodies induced by the influenza vaccine. The susceptibility to H3N8 variant by immunization-induced antibodies are directly required for risk evaluation. The development of a vaccine that stimulates the formation of targeted antibodies to fight infection is crucial in order to reduce the chance of developing viral illness.
In the current study, we created a specific vaccine using bioinformatics strategies that can target epitopes on B-cells and T-cells. Through this approach, novel epitopes of S protein H3N8 epitopes were predicted in order to create a vaccine with multiple epitopes. On the basis of toxicity, immunogenicity, and antigenicity, several epitopes were chosen, and a vaccine component with possible immunogenic qualities was created. A Multi-Epitope Vaccine was identified as a significant vaccine candidate that may aid to combat Avian Influenza infections globally.
Integrative Transcriptomic Analysis for the Identification of Novel Epilepsy Candidate Genes
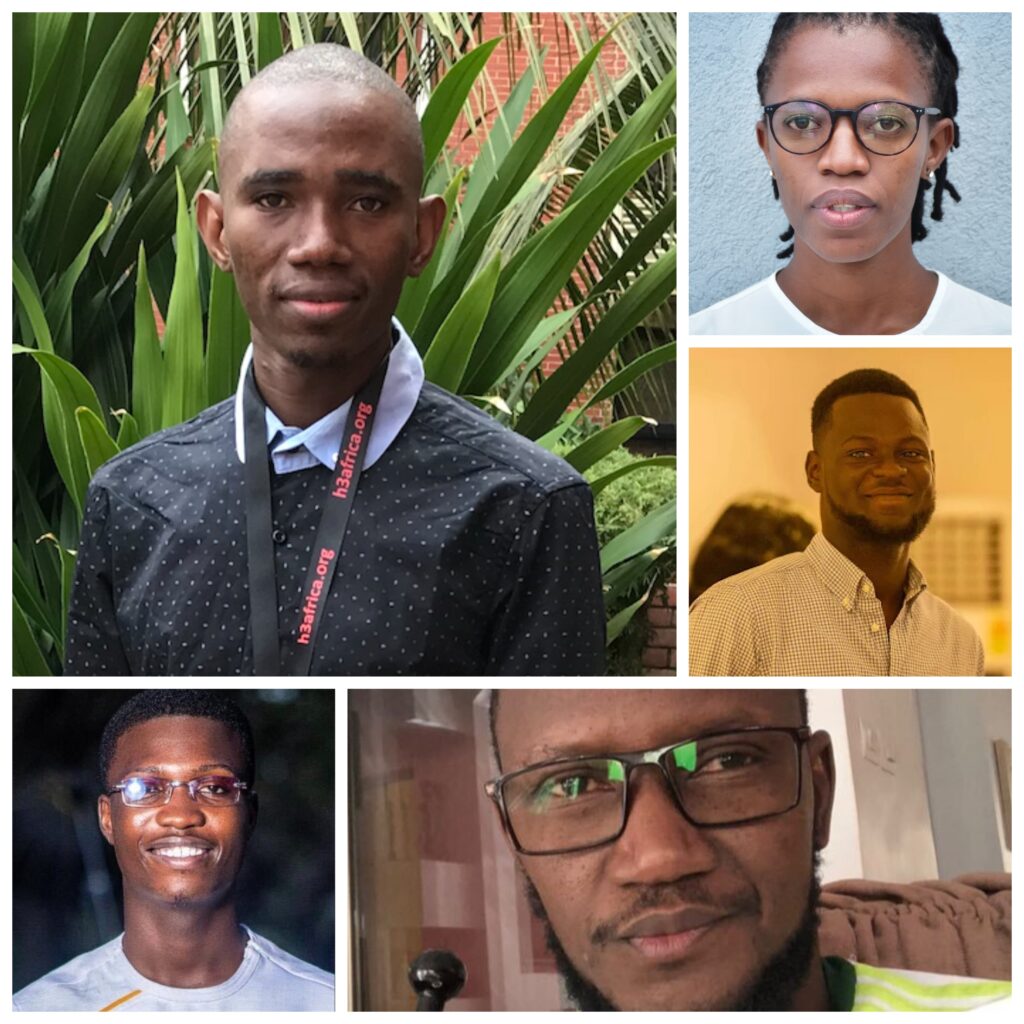
Modibo K. Goita, Diana Jepkoech, Nicholas Donkor, Pius Kwesi Sam and Lassana Coulibaly
Epilepsy, a complex neurological disorder, is influenced by various genetic factors that remain undiscovered.
In this study, we employed advanced genomic techniques to explore and uncover potential genes associated with epilepsy. Our research utilized integrative transcriptomic analysis, a method that combines data from various sources, such as gene expression patterns and molecular interactions, to gain a comprehensive understanding of gene activity in the context of epilepsy. By applying this approach, our study aims to identify previously unknown candidate genes linked to epilepsy development. These potential genes could play vital roles in the disorder’s mechanisms, paving the way for further investigation and potential therapeutic targets. Our study’s findings have the potential to advance our knowledge of epilepsy’s genetic underpinnings and contribute to the development of improved diagnostic and treatment strategies for individuals affected by the condition.
Elucidating the Contribution of Gut Pathogenic Microbes in Shaping Alzheimer’s Disease Phenotypes
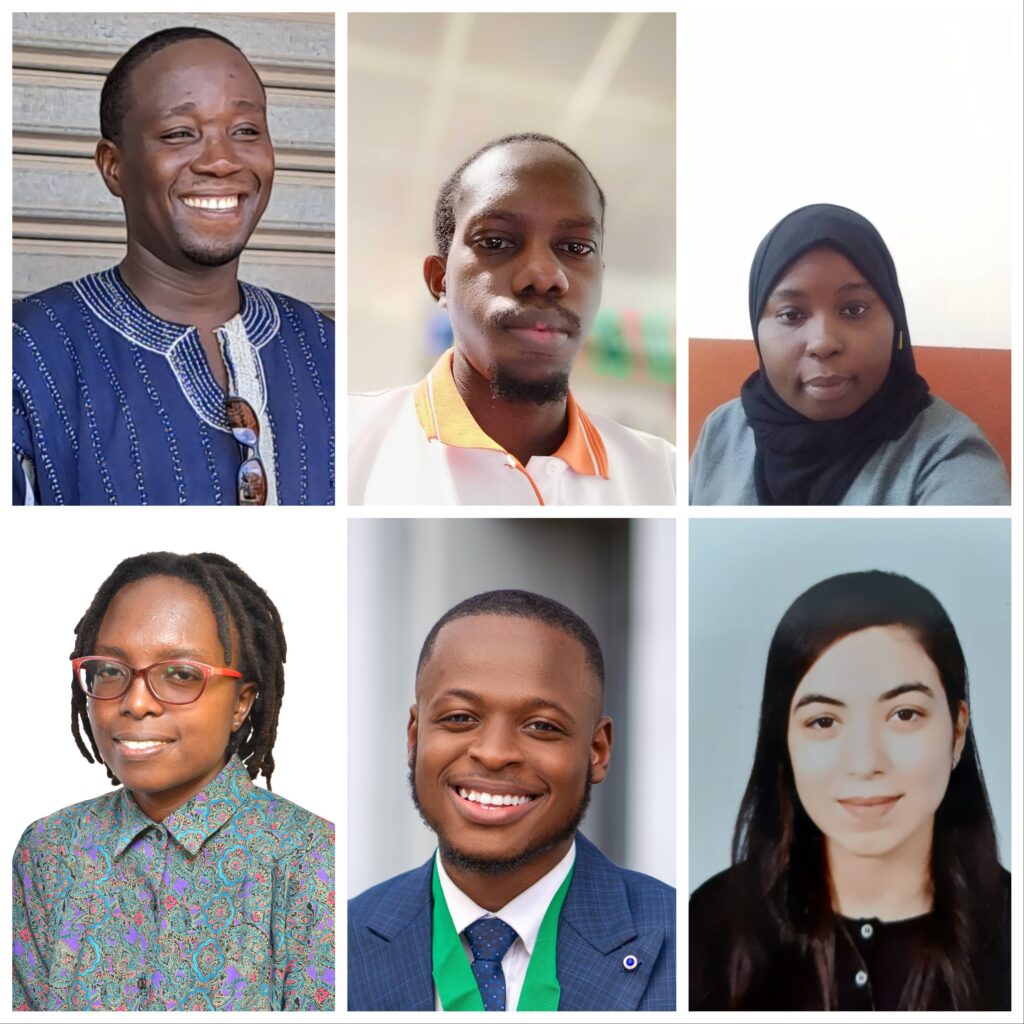
Edward Jenner Tettevi, Mark Kivumbi, Aminata Mbaye, Hildah Njoroge, Bernard Bsolodzi and Najla Zydany
Alzheimer’s disease is a global concern, demanding insights for effective interventions. Our study utilizes an existing NCBI gut-microbiome metagenomics dataset to explore the role of microbial diversity (bacteria, fungi, viruses) in Alzheimer’s phenotypes. Additionally, gender-specific microbial profiles were investigated for potential contributions to distinct Alzheimer’s expressions.
In this project, our aim was to uncover the intricate connection between microbial communities and Alzheimer’s manifestations, enriching our grasp of the gut-brain axis in neurodegenerative diseases. Notably, gender-based microbial associations could unveil sex-specific disease variations. Our study’s importance lies in deciphering how diverse microbial compositions impact Alzheimer’s complexities, holding promise for innovative disease management and personalized interventions. The identification of gender-specific microbial variations could lead to tailored treatments aligned with individual microbial profiles.
This investigation delves into microbial influences on Alzheimer’s phenotypes, utilizing NCBI’s dataset to advance our understanding of the gut-brain axis in the disease. The outcomes could shape future research and clinical strategies, harnessing the gut-brain connection to enhance Alzheimer’s management and patient outcomes.
Breast Cancer Cell Transcriptomics Reveals New Insights into the Genes and Pathways Involved in Anchorage Independence
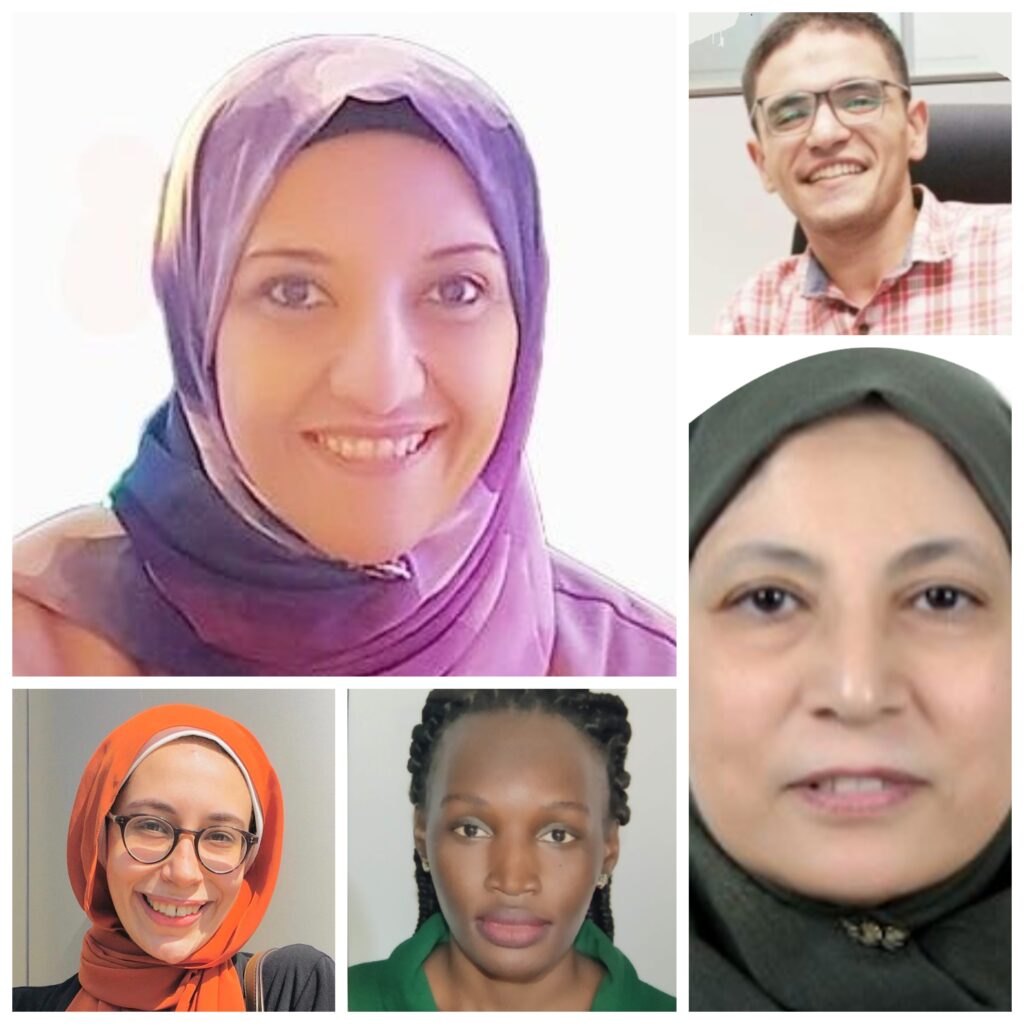
Dr. Enas A. Fouad-ElHady, Mostafa Ismail, Huda Azzam , Eva Akurut and Dr. Samar Kassem
Breast cancer is one of the most common malignancies among women throughout the world and is the major cause of most cancer-related deaths. Breast cancer cells begin inside the milk ducts and/or the milk-producing lobules of the breast. Most breast cancers are invasive. Metastasis to vital organs is identified as the principal cause of the high rate of mortality of breast cancer.
In this project, we aim to identify genes, pathways and regulatory factors implicated in anchorage independency of invasive lobular carcinoma (ILC) and invasive ductal carcinoma (IDC) and find potential therapeutic targets to abrogate metastatic dissemination. To achieve our goal, we studied the gene expression profile of the ILC and IDC breast cancer cell lines in the ultra-low attachment (ULA) suspension cultures and attached (2D) cultures through their gene expression profiles.
Bacterial Diversity in the Inflammatory Bowel Disease Metagenome: An AI-aided Approach
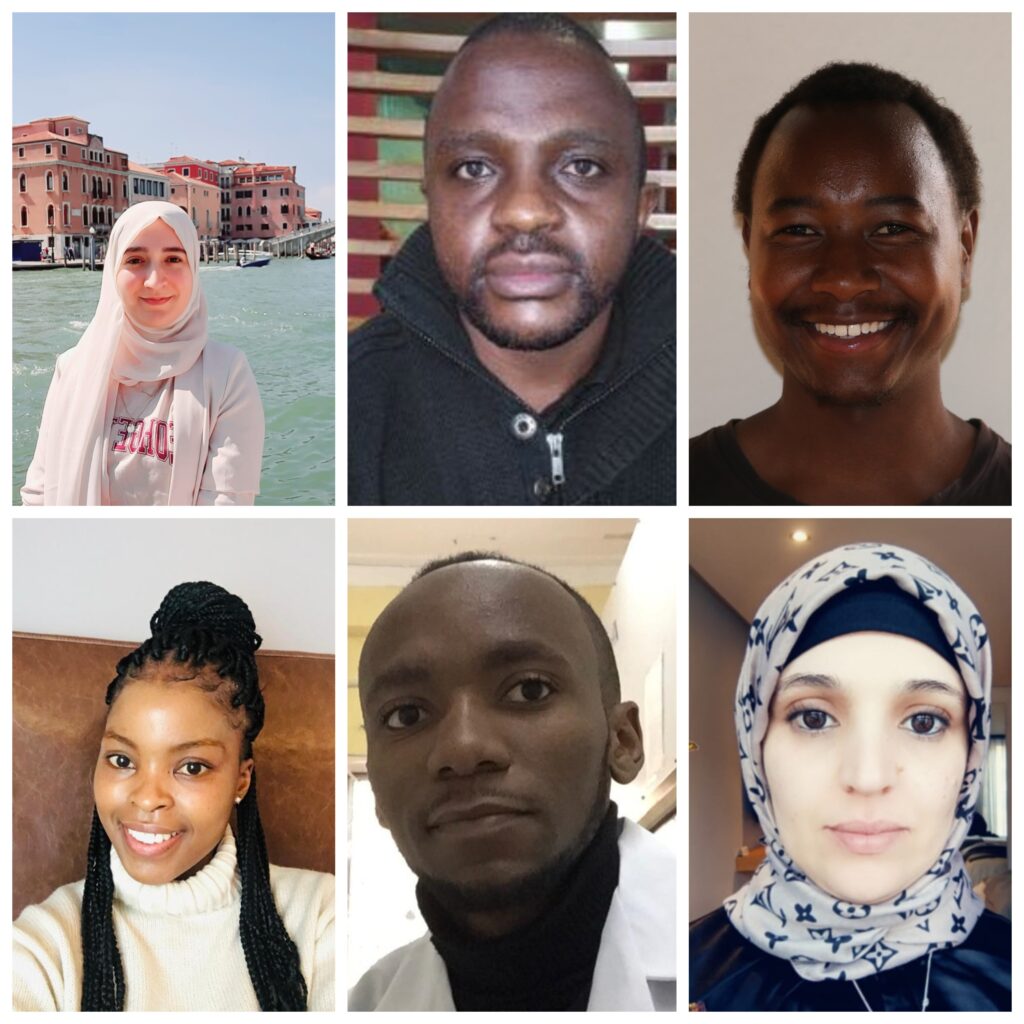
Nouhaila En najih, Gichuki Nderitu, Ephantus Wambui, Nonsikelelo Precious, Felix Lisso and Marwa Negi
The human microbiome plays an important role in maintaining human health. Recent studies have shown an association between dysbiosis of the microbiota and the development of various diseases, including inflammatory bowel disease.
Our project, we built a pipeline with seven machine learning models and applied them on inflammatory bowel disease patient’s oral metagenomic data in order to predict the presence of the disease.
In addition to prediction, we also worked on the identification of potential biomarkers associated with the disease. This initiative could facilitate the discovery of new biomarkers, the identification of potential therapeutic targets, and the development of personalized medicine for patients with inflammatory bowel disease. Using machine learning and metagenomics, our approach can provide important insights for understanding disease and developing personalized healthcare solutions.
Body Mass Index and Colorectal Adenoma Risk in Individuals of African ancestry: Two-sample Mendelian Randomization Analysis
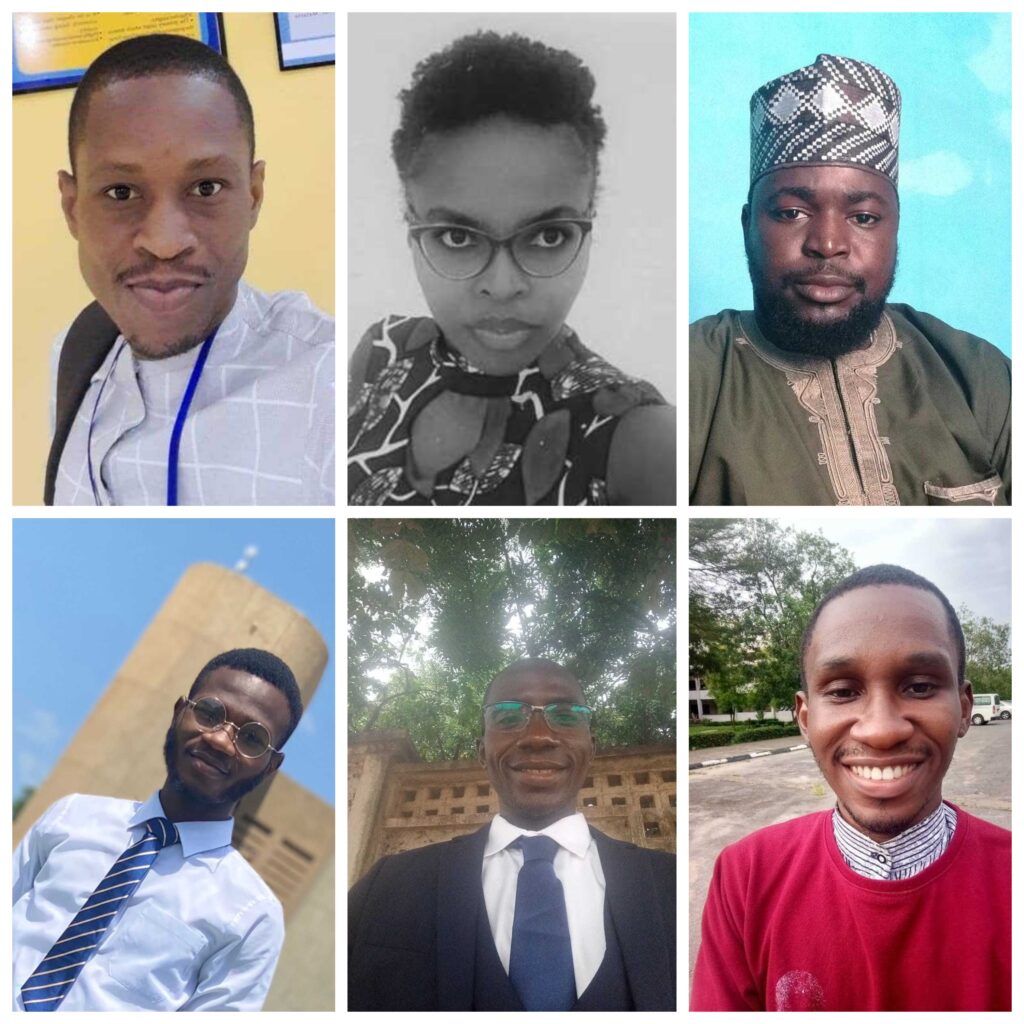
Uchechukwu Ogbodo, Pauline Kingori, Abdulrazak Sale, Andy Asante, Yusuf Eshimutu and Emmanuel Israel
Colorectal cancer (CRC) is the world’s second deadliest cancer, with 1.1 million deaths in 2018 and a projected 3 million cases and 1.6 million deaths by 2040, especially affecting native Africans due to limited screening and unhealthy lifestyles. Studies have suggested a link between diet and the onset of the disease, although observational studies do not necessarily explain the causal effect of blood lipids in the development of colorectal cancer, largely due to bias of confounding and reverse causation. Mendelian randomization, which resolves this problem, has not been sufficiently explored to understand this association in individuals of African ancestry.
To understand CRC causes, in this study, we investigated the role of serum lipids in individuals of African ancestry using multivariable two-sample Mendelian randomization (MR). Summary statistics for the exposure SNPs were obtained from the Global Lipid Genetic Consortium (GLGC) database (N = 90,400, 34.8% men) while outcome-SNPs were obtained from African-ancestry individuals in the Millions Veteran Program (MVP) (N = 23,305, 87.2% men). Our study employed a random-effects inverse variance weighted method in the primary analysis and further adjusts for pleiotropy using robust sensitivity tests.
DNA Barcoding of Medicinal Plant Species in Africa
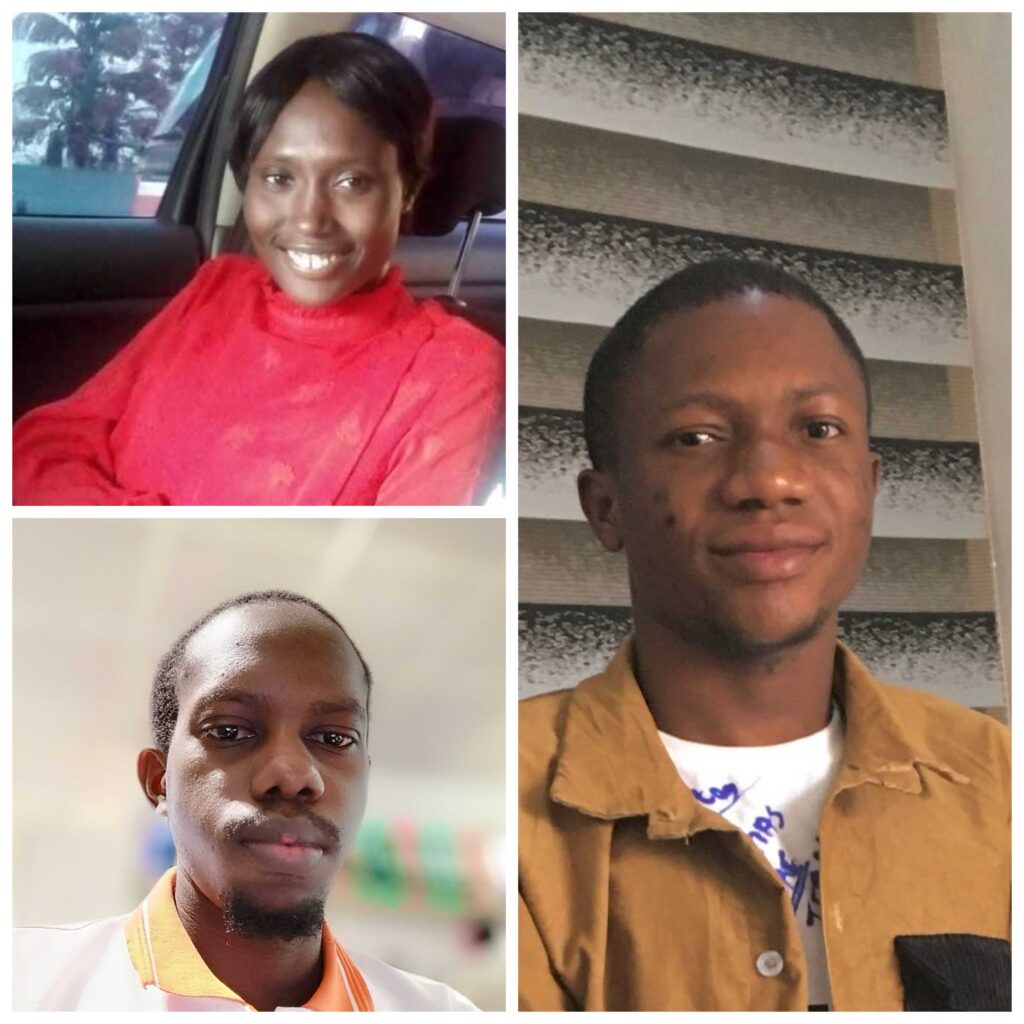
Damilola Olanipon, Mark Kivumbi and Daniel Adediran
DNA barcoding is a molecular biology technique that uses short oligonucleotide sequences called primers to identify or classify biological species. The applications of DNA barcoding range from genetic and molecular characterization to the conservation of organisms such as plants, animals, viruses, bacteria, fungi, and humans. The cytochrome oxidase (COX1) is the universal DNA barcode in animals. In plants, ITS, rbcL, matK, and psbA-trnH are the major DNA barcodes, but none has been identified as a universal barcode owing to the shortage of consensus gene regions in plant species. Research from various parts of the world and Africa in particular have identified several plant species as possessing active compounds that are medicinal in nature.
In this study, we carried out an extensive literature review of medicinal plant species in East and West Africa specifically in Nigeria, Kenya, and Uganda, and investigated the proportion of these species that have their DNA barcoded. By doing this, we hope to provide useful information on the technique of DNA barcoding; sensitive to the public on current trends and future prospects of DNA barcode reference library as a tool with application in biodiversity conservation, molecular systematics, and forensic examination in cases of adulterated medicinal plant formulations.
Prediction of novel antimicrobial resistant genes in Acetinobacter baumannii using Machine Learning, Homology Modelling and Molecular Docking
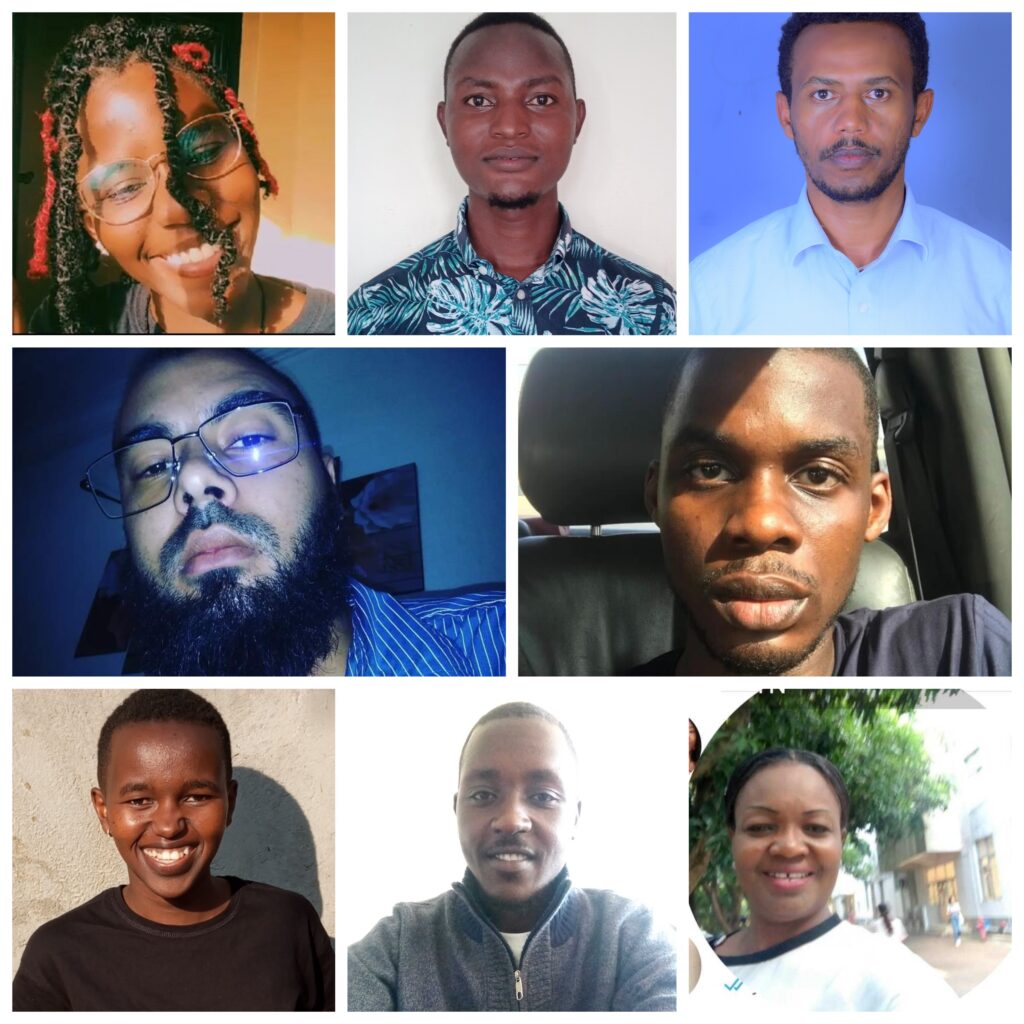
Vanessa Natasha Onyonyi, Henry Ndugwa, Sisay Teka Degechisa, Firas Zemzem, Jude Alao, Parcelli Jepchirchir, Jimmy Nkaiwuatei and Florence Mbaoji
The emergence of increased antimicrobial resistance (AMR) in Acinetobacter baumannii has exacerbated the mortality and morbidity rates globally. This pathogen’s genome plasticity at evading antibiotics, particularly in healthcare settings, disrupts conventional treatment methods.
In this study, we developed a machine learning model that predicts novel antimicrobial genes and validates the output with homology modelling and molecular docking as an initiative for combating AMR. The selection of this approach was done in order to assist in treatment choices, maximise antibiotic usage, and bolsters outbreak responses. Additionally, it contributes to understanding AMR evolution and facilitates personalised medicine. Ultimately, this innovative endeavour strengthens the battle against AMR and secures antibiotic efficacy in the face of this pressing global health crisis.