Omics codeathon is an established event where life scientists work on research projects. It is held twice a year and led by Olaitan I. Awe (Ph.D.), the current training officer for the African Society for Bioinformatics and Computational Biology (ASBCB).
The October 2024 omics codeathon was virtual.
The primary aim of the omics codeathon is to use omic sequences and bioinformatics to advance the understanding of the biology of model organisms and pathogens to ultimately improve human health. Our research projects typically use human, cellular, cancer and pathogen genomics to investigate the molecular mechanisms of mendelian disorders and complex traits. By using different omics approaches, we are interested in trying to understand how diseases work at the molecular level.
Codeathon participants and applicants come from across the globe, including South Africa, Kenya, Nigeria, Libya, Tunisia, Algeria, Zimbabwe, Morocco, Egypt, Senegal, Mali, Ghana, Uganda, Mozambique, Tanzania, Botswana, Burkina Faso, Gambia, Benin Republic, Ethiopia, Sudan, Malawi, Zambia, Cameroon, Conakry-Guinea, Democratic Republic of Congo, Namibia, Denmark, Malaysia, Turkey, Indonesia, Pakistan, Portugal, Brazil, Poland, Bangladesh, South Korea, Saudi Arabia, Dubai / United Arab Emirates, India, Taiwan, China, Sweden, Finland, Germany, France, Ireland, Spain, Australia, Philippines, Mexico, United States, United Kingdom and Canada.
The October 2024 codeathon projects were in these categories; Bulk Transcriptomics, Pathogen Genomics, Metagenomics, Human Genomic Variation, Pipeline Development, Biomarker Discovery, Cheminformatics, Clinical Applications, Drug and Vaccine Design, Drug Resistance, Population Genomics, Antimicrobial Resistance (AMR), Genome Wide Association Studies, Mendelian Randomisation, Oncology, Structural Bioinformatics, DNA Methylation, Plant Genomics, Metabolomics, Single-cell Transcriptomics, Epigenomics and Machine Learning.
Omics Codeathon Supporters:
- BioData Sage
- National Institutes of Health, Office of Data Science Strategy
Projects
Title
Team
Project Description
ProStruc: A Python-based Tool for Homology Modeling and 3D Structure Prediction
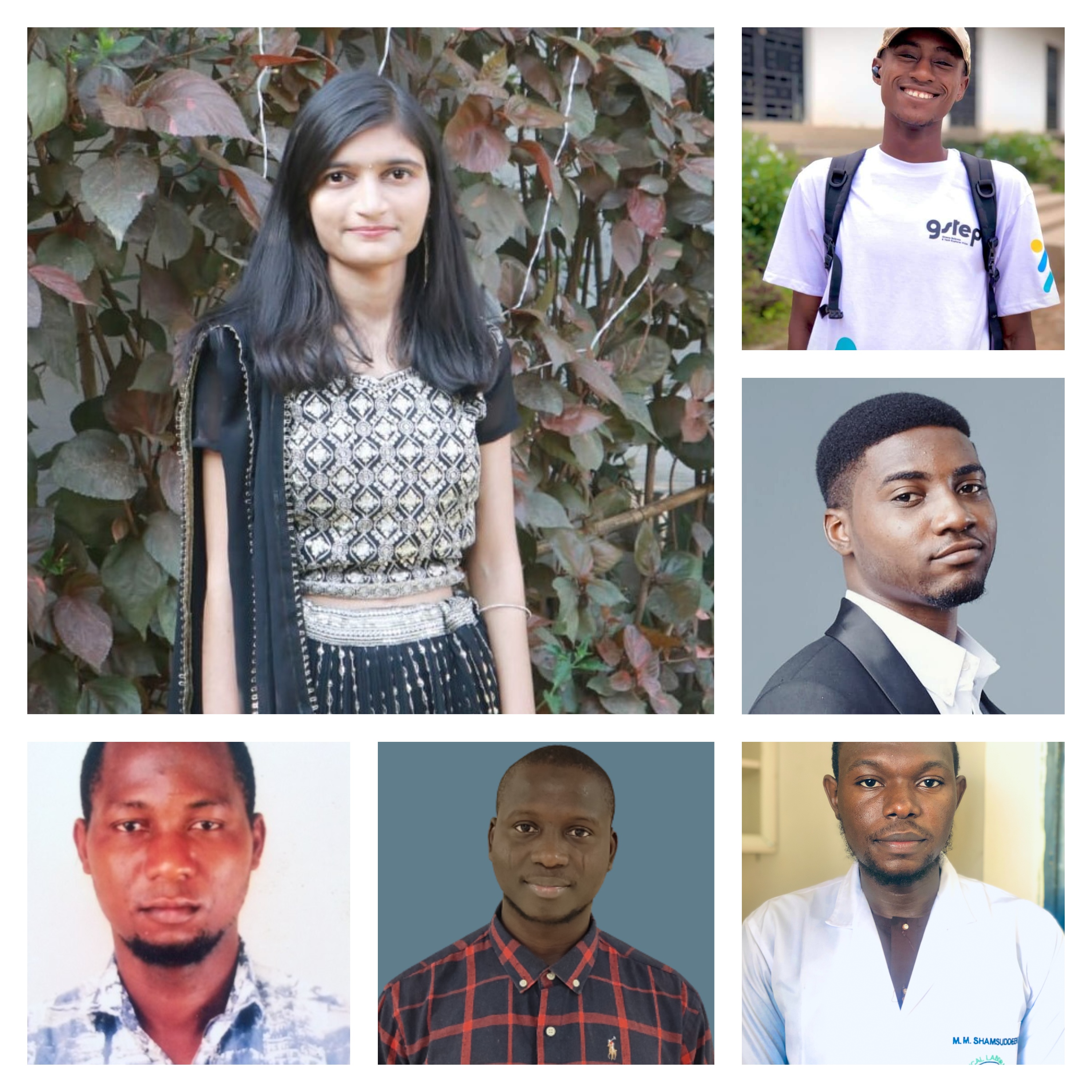
Shivani V. Pawar, Wilson Sena, Nigel Dolling, Toheeb Jumah, Abdulwasiu Tiamiyu, and Musa Muhammad Shamsudeen
ProStruc is an innovative project aimed at making protein structure prediction accessible and efficient for researchers of all levels. This open-source, automated homology modelling tool integrates advanced computational techniques into a user-friendly platform designed to streamline the prediction of three-dimensional protein structures. It uses advanced computational techniques, including biopython for sequence alignment and BLAST searches, to streamline the prediction of three-dimensional protein structures. ProStruc automates the identification of suitable template structures from the Protein Data Bank (PDB) and uses MODELLER and the PROMOD3 algorithm as its homology modelling engines. This ensures accurate and reliable predictions based on alignment scores and customizable parameters. The tool features a modular design, allowing integration with additional prediction and validation tools, and supports both single and multiple sequence inputs for high-throughput research. ProStruc’s intuitive graphical user interface (GUI) simplifies the entire workflow, from sequence alignment to preliminary structure validation, making it accessible to researchers with varying levels of expertise. By promoting collaboration and continuous improvement through its open-source nature, ProStruc aims to advance protein structure prediction, empower researchers, and enhance our understanding of protein functions and interactions.
Comparative Transcriptomic Analysis of UHRF1 Knockout in Different Cancer Cell Lines
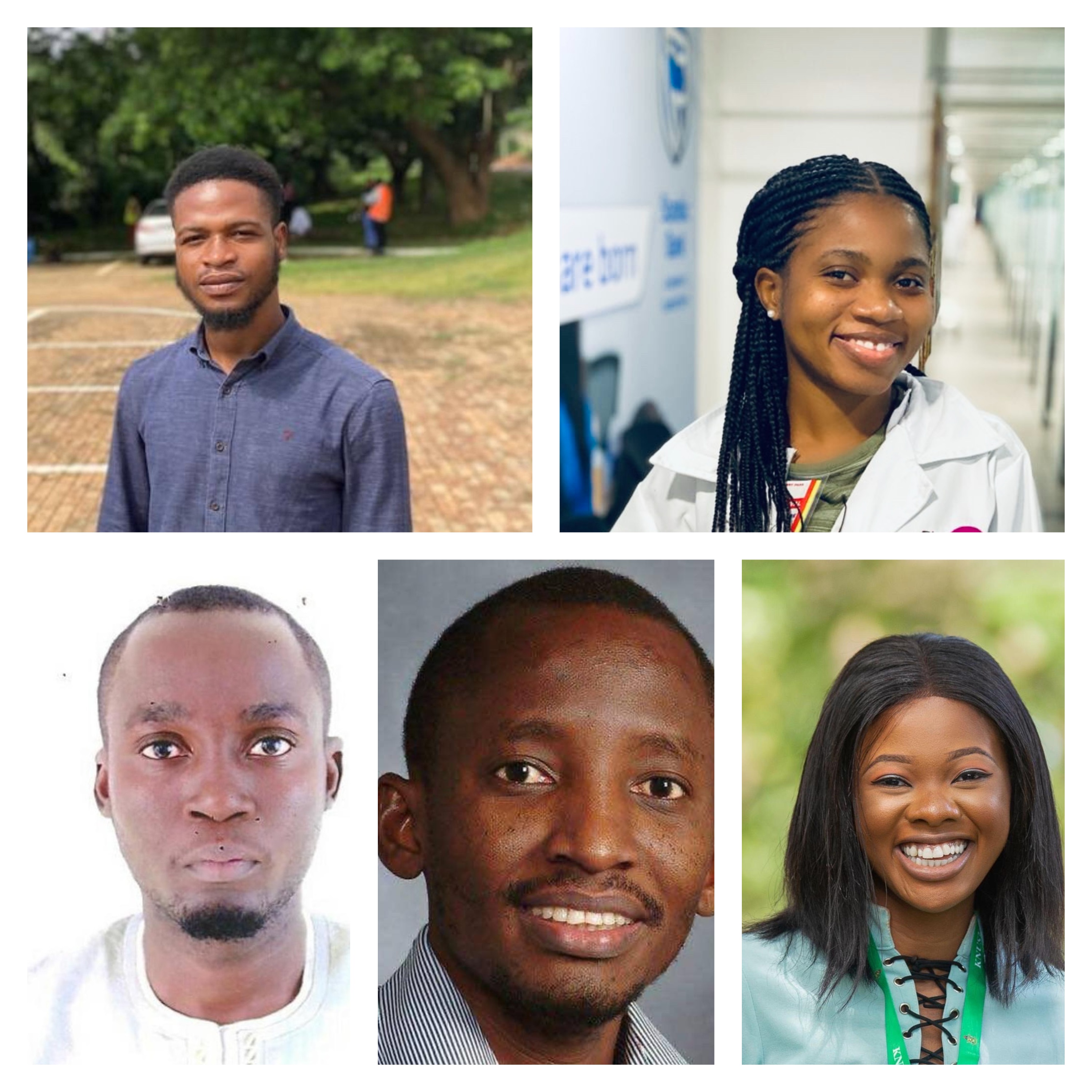
Jonathan Kalami, Miriam E.L. Gakpey, Benthai Benjamin, Benson Kidenya and Sala Kotochi
In this project, we did an in-silico study to perform a comparative transcriptomic analysis of UHRF1 knockout in four distinct and most common human cancer types namely; breast cancer, retinoblastoma, acute myeloid leukemia and acute monocytic leukemia. We analyzed publicly available datasets from the Gene Expression Omnibus (GEO) containing RNA sequencing data on cancer cell lines of breast cancer (MCF-7), retinoblastoma (Y79), acute myeloid leukemia (Kasumi-1) and acute monocytic leukemia (THP-1). We performed differential gene expression and gene set enrichment analysis to assess differentially expressed genes (DEGs) and pathway dysregulation resulting from UHRF1 gene loss.
Understanding the differential impacts of UHRF1 across different cancer types, along with common gene targets and shared dysregulated pathways offers better insights into the mechanisms underlying UHRF1-mediated gene regulation, which is the key towards suggesting important biological processes that may be targeted for therapeutic intervention across various cancer types. Furthermore, it provides biomarkers for predicting the risk of acquiring cancers.
Plant-pathogen Interactions between Xanthomonas oryzae and its Host
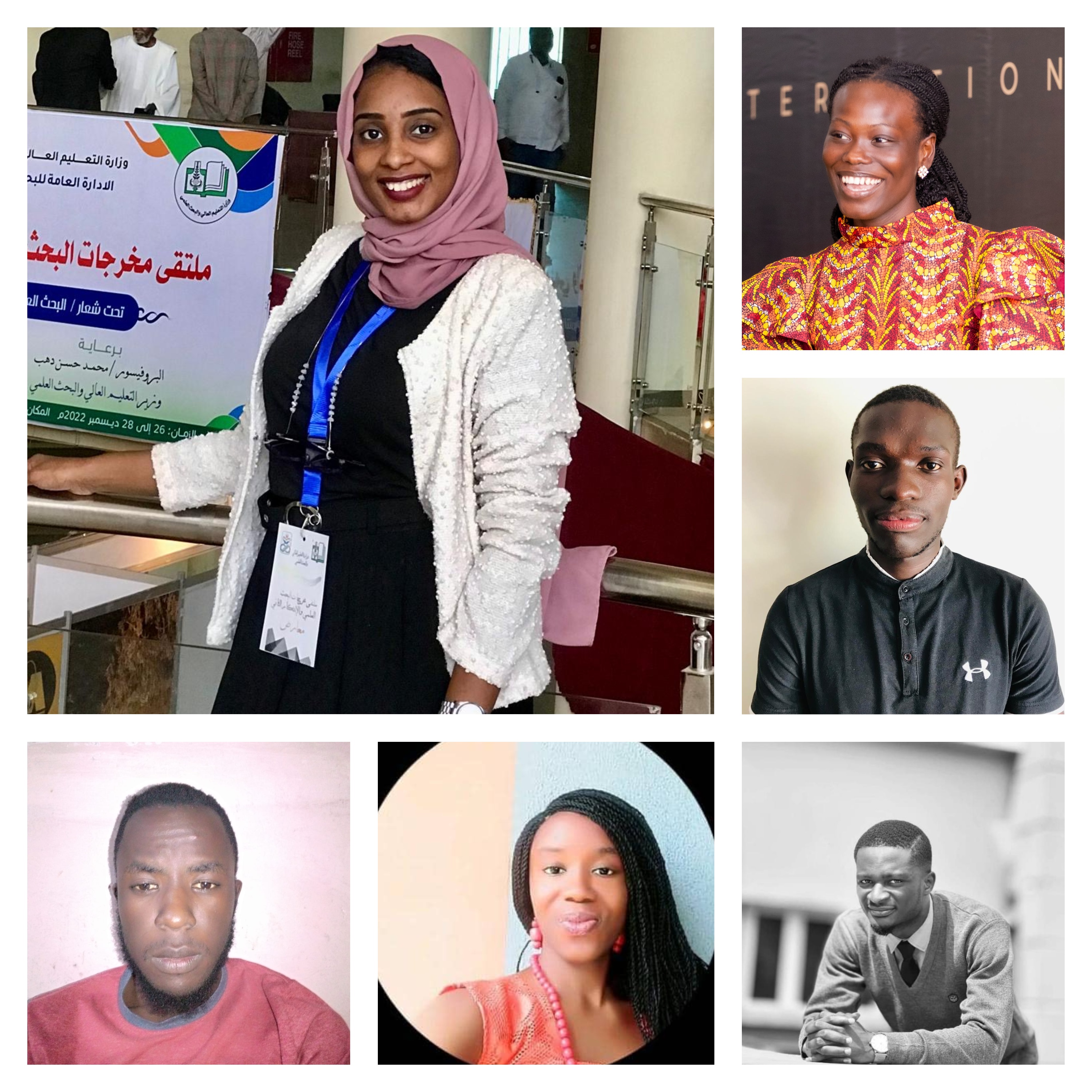
Khadija Elamin, Jessica Arthur, Paul Ajwang, Isaya Odongo, Akachukwu Onwuka and Samuel Owusu-Ansah
Rice is a vital food source for half of the world’s population, but its growth is severely affected by bacterial leaf blight, a disease caused by Xanthomonas oryzae pv. oryzae (Xoo). This pathogen significantly reduces both the yield and quality of rice. Despite ongoing research, a comprehensive understanding of the core set of rice genes and microRNAs (miRNAs) crucial for defense against Xoo infection is lacking. Our research aims to identify a core set of genes and microRNAs (miRNAs) that play crucial roles in rice defence against Xoo. We uncover the regulatory networks that control these responses by analysing time-series RNAseq datasets using a state-of-the-art machine learning approach. We also provide a reproducible pipeline for further studies of this nature.
Machine Learning and Molecular Docking Prediction of Potential Inhibitors against Dengue Virus
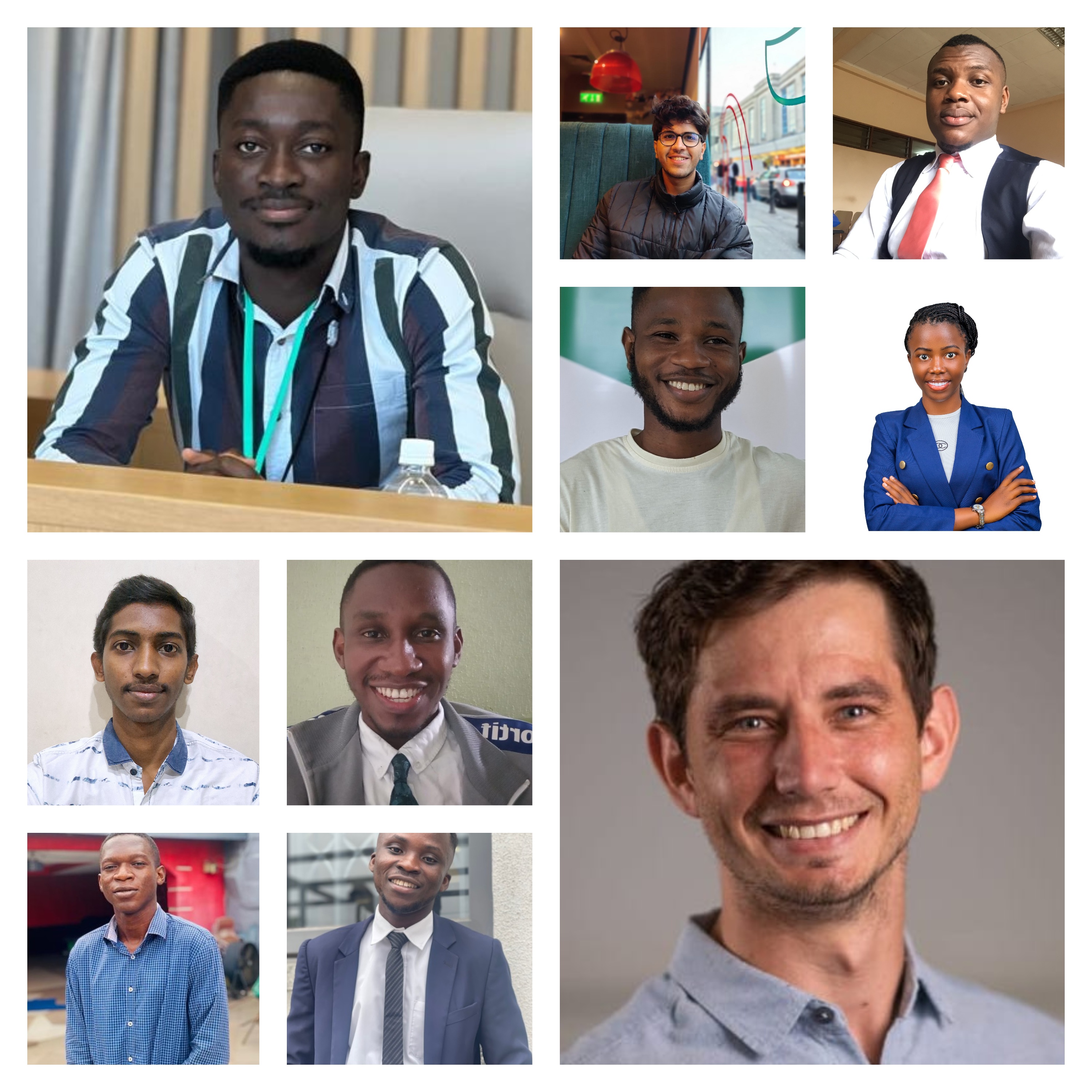
George Hanson, Andy Asante, Emmanuel Nsedu, Hem Bondarwad, Joseph Adams, Kepgang Daveson Innocento Brank, Lewis Tem, Soham Amod Shirolkar, Maureen Kisaakye and Luke Zondagh
The most common viral hemorrhagic fever spread by mosquitoes is caused by the dengue virus (DENV), a member of the Flaviviridae family, transmitted by Aedes spp. in tropical and subtropical regions. The prevalence of dengue remains a significant global threat to public health with millions of infections and mortality reported annually due to the lack of effective treatment regimens. However, the availability of well-curated compound databases, structural information on DENV, and the advancement of bioinformatics tools and Machine Learning offer unprecedented opportunities to predict novel compounds for the inhibition of DENV. A key target for the development of an antiviral drug that prevents conformational changes and interferes with membrane fusion is the envelope protein.
In this study, we predicted novel inhibitory compounds against the dengue virus by utilizing in-silico pipelines that integrate various machine-learning models and molecular docking simulations. The research will build and evaluate the performance of different models to identify the most accurate and reliable model for compound prediction, using data derived from natural resources such as plants as they contain phytochemicals with desirable therapeutic effects. Additionally, molecular dynamics simulations will be employed to characterize the binding mechanisms of the predicted lead compounds against the envelope protein of DENV.
Genomic Landscape of HIV Mutation in Nigeria: Identifying Mutations and Conserved Regions for Targeted Therapeutics
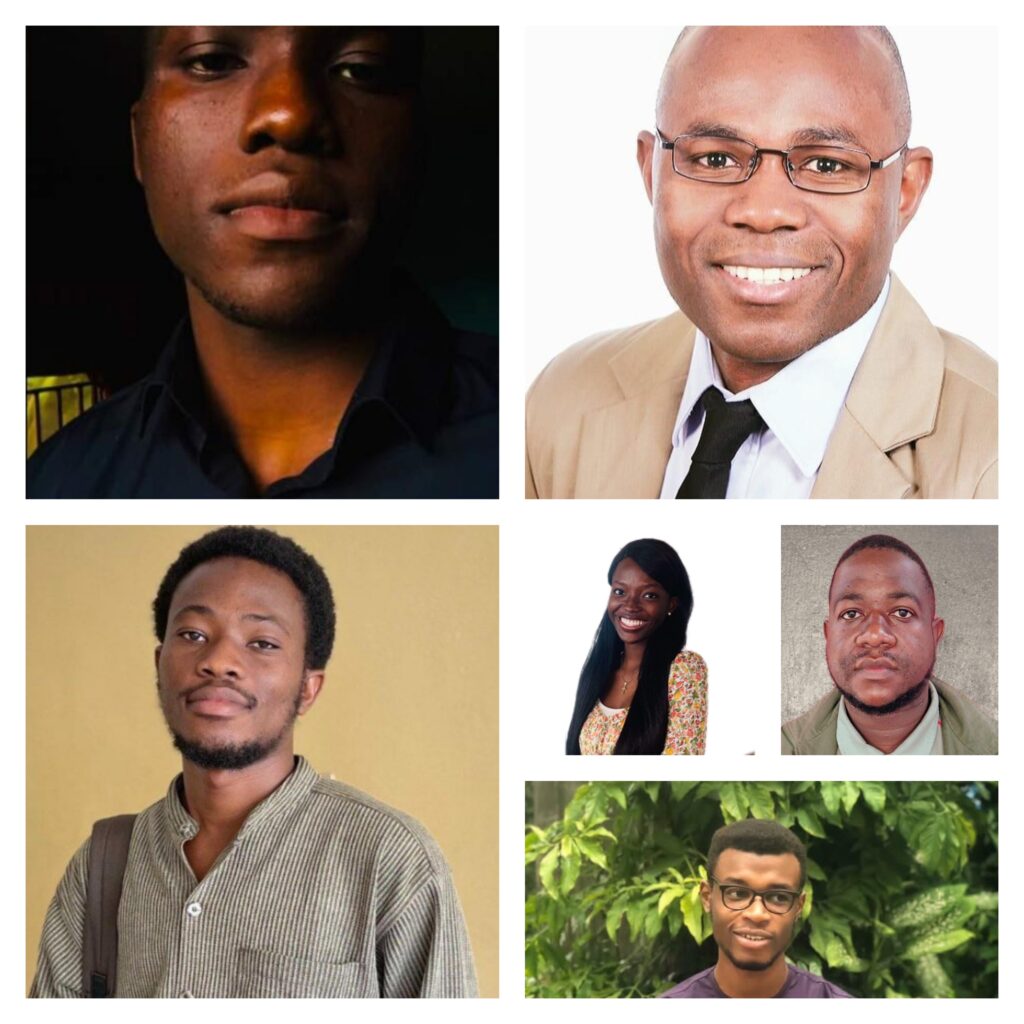
Halleluyah Darasimi Oludele, Koney Shardow Abdul Latif, Julien A. Nguinkal, Maame Esi Annor-Apaflo, Phazha Bushe Baeti and Jonas Ibekwe Paul
Mutations leading to HIV Drug Resistance pose a great barrier to the use of existing therapies and also development of new ones (eg. antisense therapy). Advanced countries have a more robust system of determining drug resistance which has helped with the development of newer therapies. However, the knowledge of the mutation patterns and their effects in Nigeria needs more light.
In this project, through the use of high quality sequence data and bioinformatics workflows, we investigated the genetic diversity of HIV in Nigeria to uncover critical mutations associated with drug resistance and to identify conserved regions across various HIV strains. By analysing assembled RNA genomes from Nigerian HIV samples, the project aims to pinpoint commonly mutated genes, assess gene variations, and locate conserved regions crucial for potential therapeutic interventions. This will help use current therapies effectively and also help in the development of novel therapies for this purpose, in this case, antisense therapy. Using a combination of advanced bioinformatics tools, including the nf-core/viralrecon pipeline, BWA, and SnpEff, the study will perform comprehensive quality control, alignment, and variant calling. Data will be cross-referenced with known drug resistance mutations to inform targeted therapeutic strategies. Additionally, phylogenetic analysis will reveal evolutionary patterns, while visualisation tools will aid in presenting mutation frequencies and conserved regions. The findings will enhance understanding of HIV drug resistance in Nigeria and guide the development of effective, region-specific treatments.
Investigating Structural Variants in Chronic Kidney Disease: A Bioinformatics Analysis
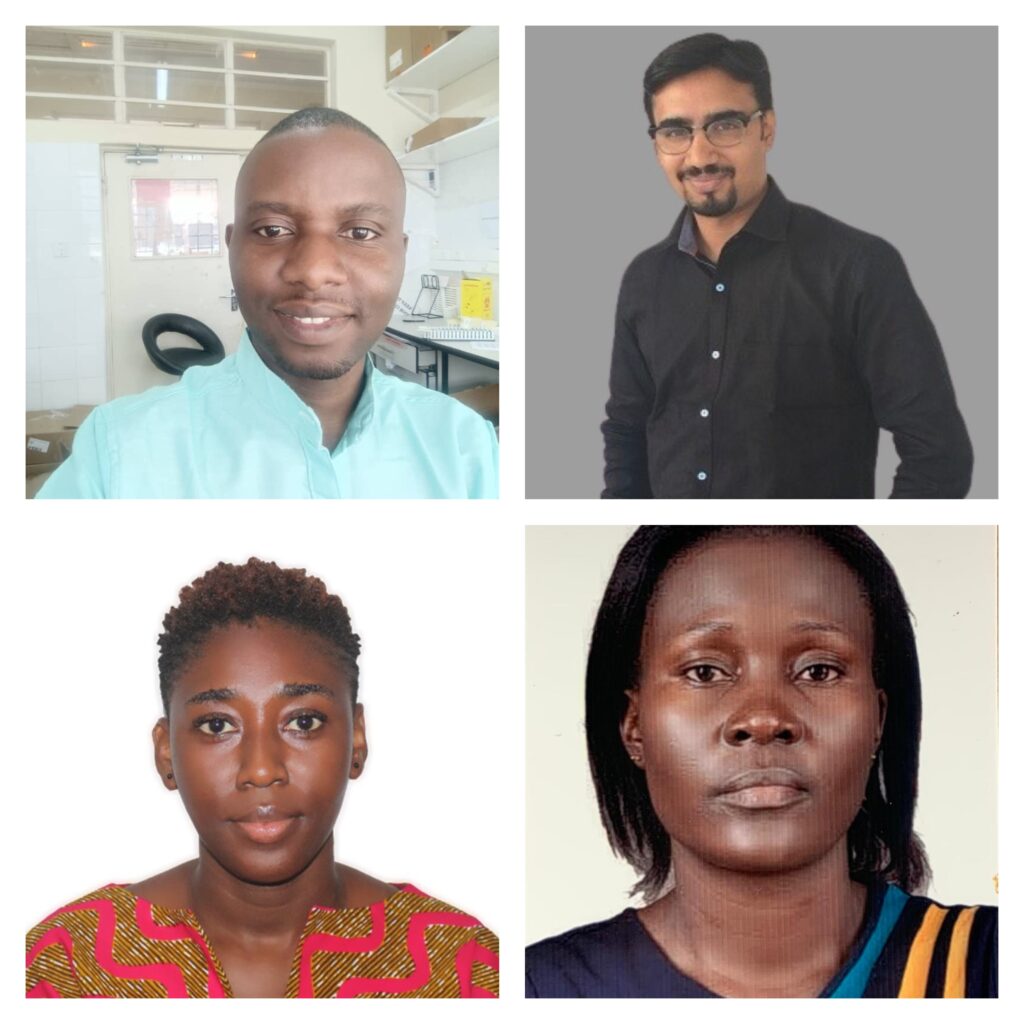
Chimenya Ntweya, Mandar Bedse, Nana Yaa Achiaa Karikari Agyemang and Aweco Evelyn Atim
Chronic Kidney Disease (CKD) poses a significant health burden worldwide, affecting millions of individuals and contributing to morbidity and mortality. Chronic kidney disease (CKD) patients have many affected physiological pathways. Variations in the genes that regulate these pathways may affect the incidence and predisposition to this disease. Although the etiology of CKD is multifaceted, recent advancements in genomic research have highlighted the role of structural variants (SVs) in the genetic landscape underlying CKD susceptibility. Structural Variants (SVs) represent essential forms of genetic variation and are associated with various CKD phenotypes. This project aimed to comprehensively investigate SVs associated with CKD by employing cutting-edge genomic techniques and computational analyses. In this study, we sought to elucidate the spectrum of SVs implicated in CKD pathogenesis by integrating next-generation sequencing technologies, bioinformatics algorithms, and clinical data mining.
We analysed whole exome data of multiple sclerosis patients and controls in order to explore the molecular mechanisms underlying causal variants and to identify variants that might escape detection by conventional genetic studies.
Single-cell Transcriptomic Profiling of Drug-Resistant Epilepsy Patients for Biomarker Discovery
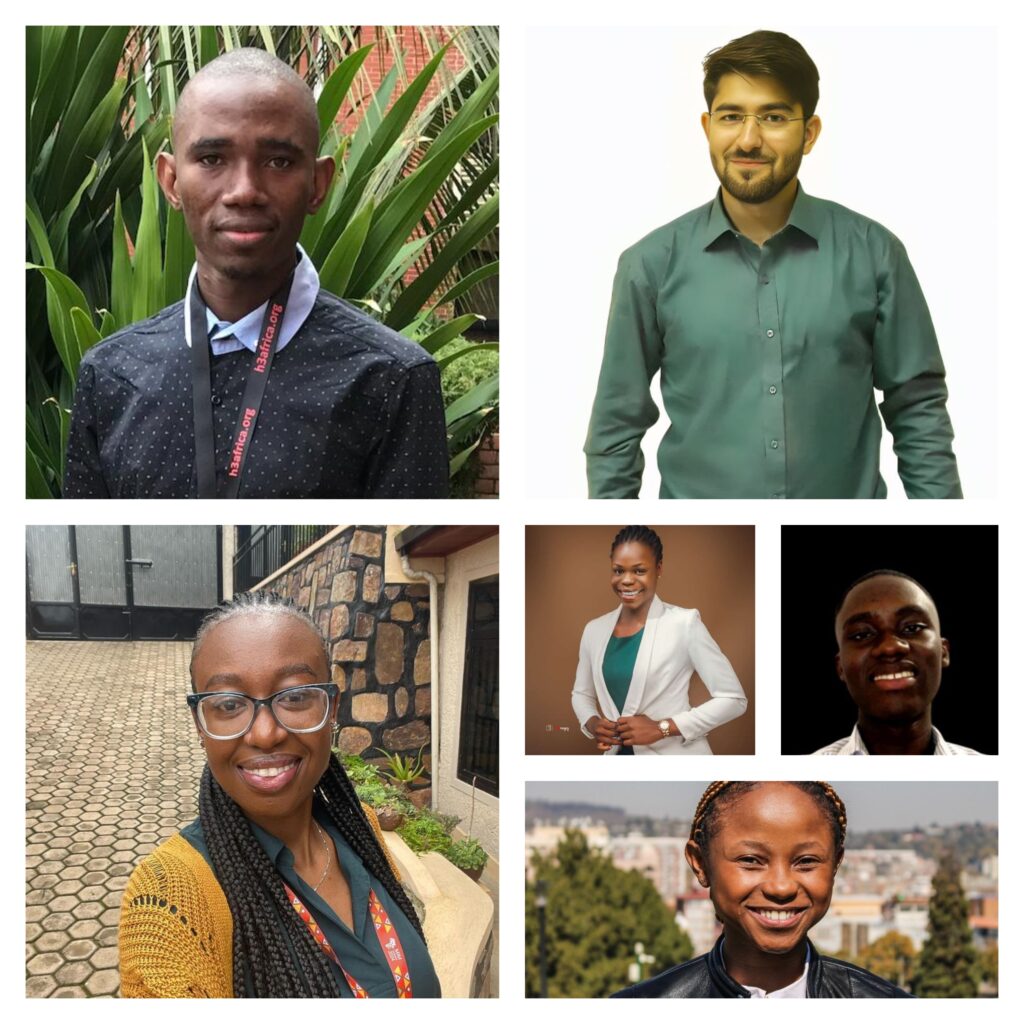
Modibo K. Goita, Shamim Osata, Muhammad Uzair Khan, Celestina Oluwaseun Olafusi, Gerald Duah Adu-Broni and Maboletso Letsoalo
Epilepsy, a complex neurological disorder characterized by recurrent seizures, affects approximately 50-70 million individuals globally. Despite significant advancements in treatment, a substantial number of patients remain resistant to existing therapies, highlighting the urgent need for new approaches to enhance diagnosis and treatment strategies. Single-cell transcriptomics, a powerful technique that allows for the exploration of the cellular and molecular landscapes of tissues at an unprecedented resolution, holds promise in addressing this challenge. In this study, we aim to uncover novel biomarkers associated with drug-resistant epilepsy by examining gene expression patterns in cells from surgically resected epileptic tissues of refractory patients. Utilizing advanced single-cell RNA sequencing (scRNA-seq) technology, we seek to gain deeper insights into the cellular mechanisms underlying epilepsy. Our findings have the potential to significantly advance the understanding of epilepsy, paving the way for the discovery of novel biomarkers and the development of targeted therapies.
Cell Lineage and Trajectory Analysis for Single-Cell RNA Sequencing with Rare Cell Populations in Multiple Sclerosis Patients Data
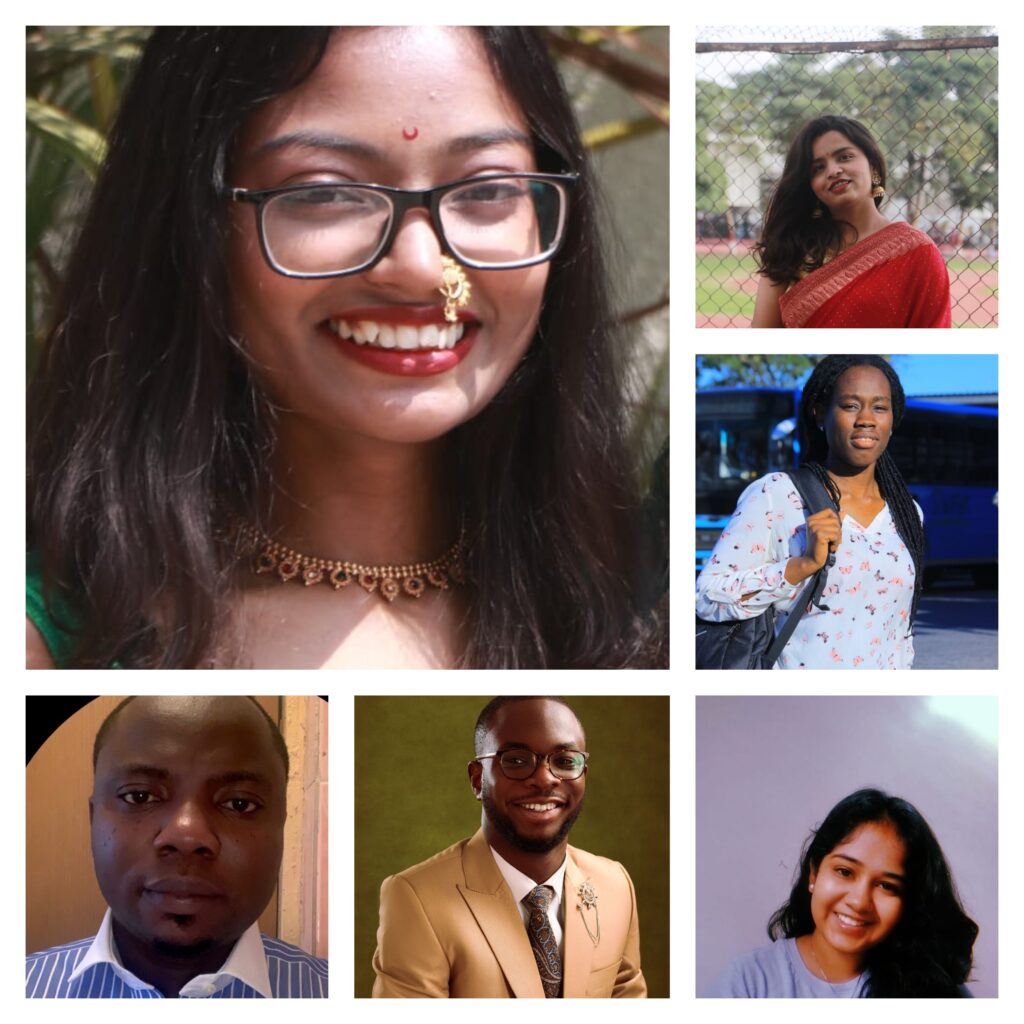
Prachi Tayade, Adetayo Aborisade, Felix Oluwasegun Ishabiyi, Hwenude Judicaelle Chance Gountin, Vaishanavi Yadav and Yamini Sudame
Multiple sclerosis (MS) is a neurodegenerative disease affecting the central and peripheral nervous systems, leading to significant disability. With around 2.8 million people affected worldwide, MS is the most common cause of non-traumatic neurological disability in young people, particularly women, who are diagnosed three times more frequently than men. Despite extensive research, the cellular mechanisms of MS remain poorly understood. Recent studies highlight the crucial role of T lymphocytes in MS pathogenesis, where they invade the central nervous system, destroying the myelin sheath. This immune response also involves B cells and microglia, amplifying the inflammatory cascade.
However, gaps remain in understanding the dynamics of rare immune cell populations in MS. Single-cell RNA sequencing (scRNA-Seq) has emerged as a transformative technology for characterizing cellular diversity at an unprecedented resolution, especially for rare cell populations. In this project, we aim to use scRNA-Seq to investigate the lineage relationships and developmental trajectories of rare immune cell populations in MS, thereby enhancing the understanding and identification of novel therapeutic targets for future treatment strategies.
Mapping the Epigenomic Landscape in Alzheimer’s Disease
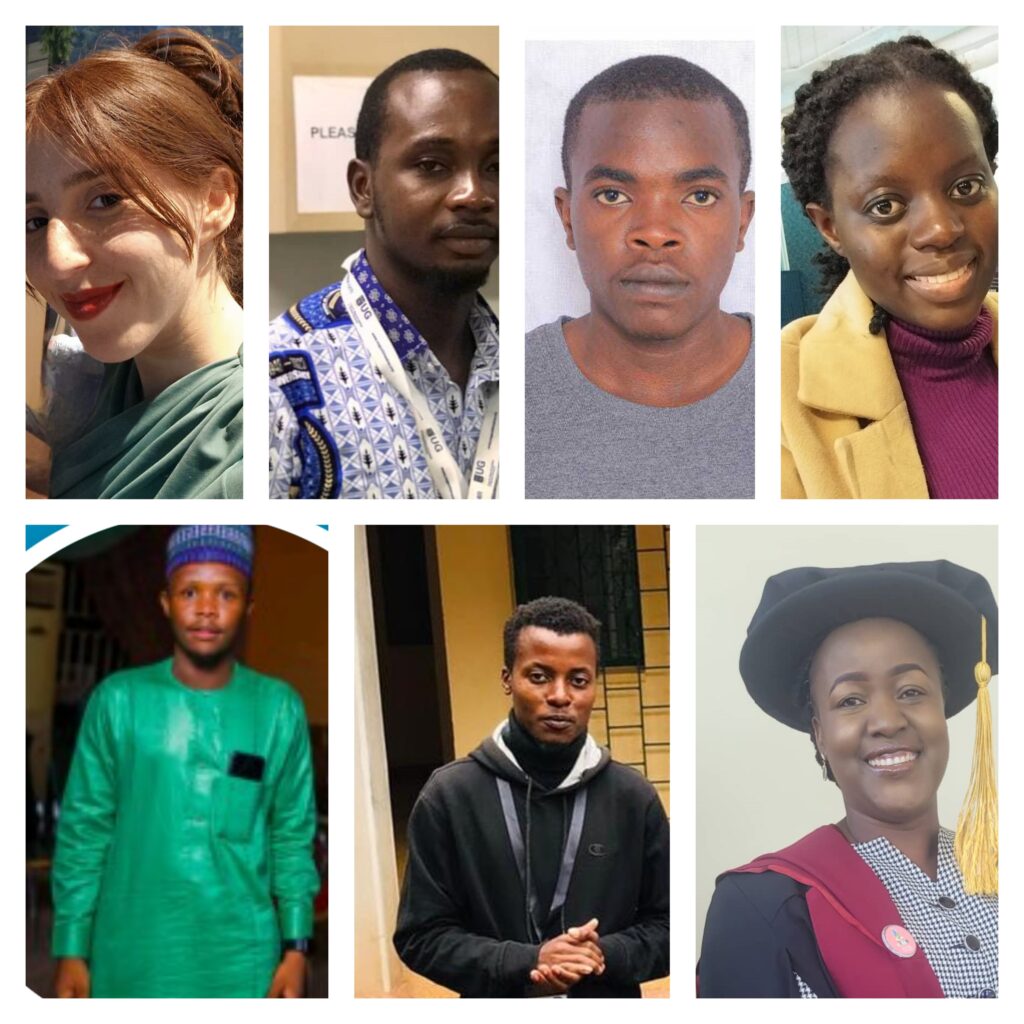
Nour ElHouda Barhoumi, Prince Fordjour, Samuel Mutwiri, Veronica Recheal Wokibula, Mubarak Garba Bala, James Kariuki and Hortensia Gaspar Nondoli
Alzheimer’s disease (AD) presents a complex interplay of genetic, epigenetic, and environmental factors. Our longitudinal study utilized datasets taken from the public repository GEO (Gene Expression Omnibus) to unravel the sex-specific epigenomic changes associated with Alzheimer’s disease. Utilizing high-throughput RNA sequencing, we meticulously profiled gene expression across various ages and sexes, providing unprecedented insights into the molecular underpinnings of AD.
Key findings revealed that males exhibited an accelerated upregulation of immune-related genes, highlighting significant sex differences in inflammatory responses. These results underscored the critical role of chronic inflammation and complement activation in exacerbating Alzheimer’s pathology. The comprehensive dataset mirrored postmortem brain analyses from Alzheimer’s patients, serving as a valuable resource for developing targeted therapeutic strategies and biomarkers.
We explored the epigenomic landscape of Alzheimer’s disease, aiming to bridge the gap between biological aging and disease pathogenesis, offering new hope for combating this debilitating condition.
A Python Package for Rhinovirus Genotyping
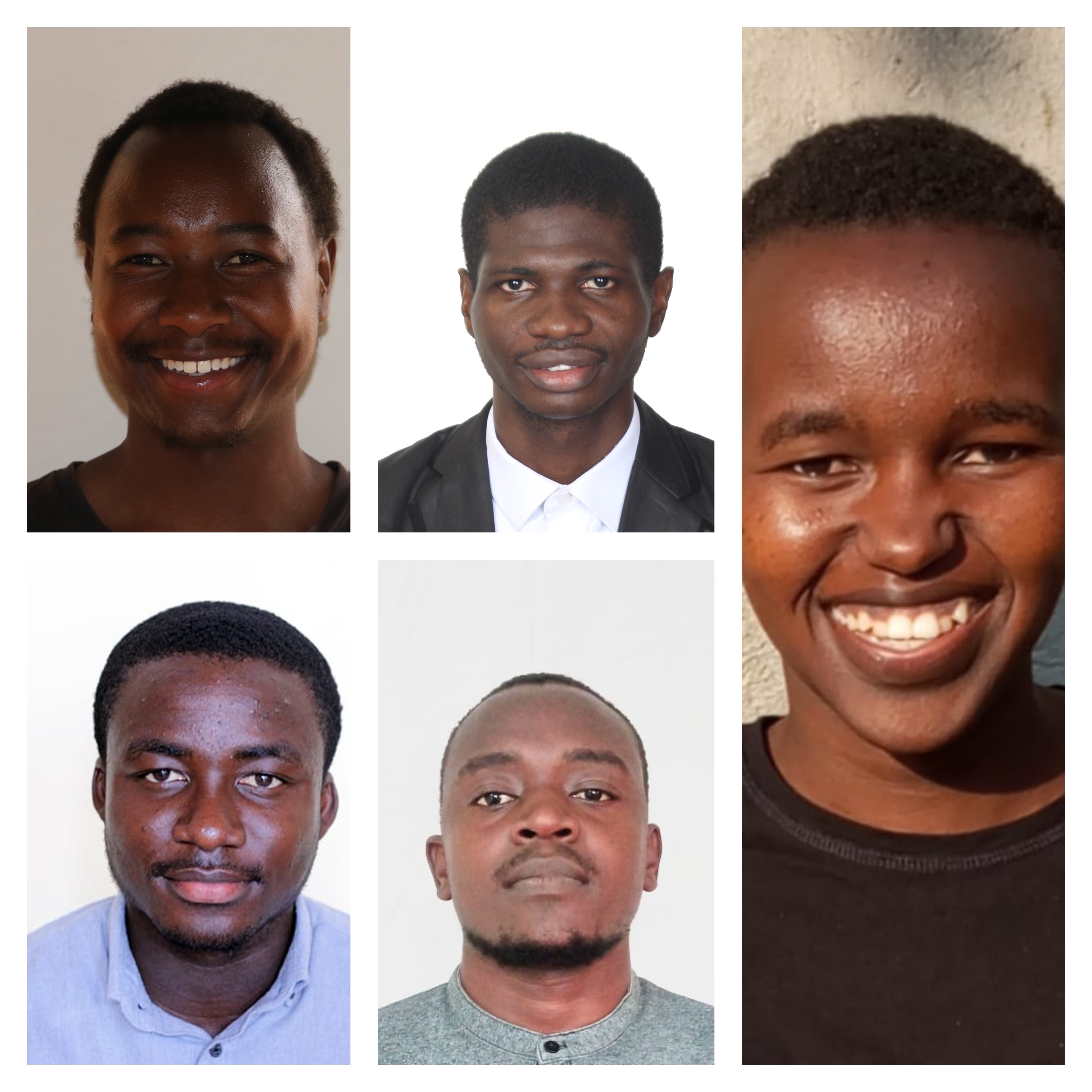
Ephantus Wambui, Daniel Okoro, Andrew Acheampong, Manase Aloo and Parcelli Jepchirchir
Among the major causes of human respiratory infections, rhinovirus causes around 50% of all the year-round cases of common cold. This virus belongs to the Enterovirus genus in the Picornaviridae family. RV-A, RV-B, and RV-C are the three species under the genus Rhinovirus, each having about 169 different genotypes. Rhinoviruses are positive-sense, non-enveloped RNA viruses with approximately 7.2 kilobases long genome. The genome codes for seven non-structural proteins and four structural proteins—VP1, VP2, VP3, and VP4—which are part of the genome encoding viral replication and host infection mechanisms. Genotyping of Rhinovirus, until now, is done manually, while an R software package, rhinotypeR, allows for fully automated genotyping based on the VP4 region.
In this study, we developed the Python rhinovirus genotyping package which brings out VP1 and VP4 regions that aim to improve acuity in genotyping. The VP1 region has been tested to display better acuity in genotypic identification, while the VP4/2 region ensures compatibility under one universal amplification protocol for rapid genotyping.
Bacgen: An Advanced Pipeline for Antimicrobial Resistance Profiling and Network Analysis in Bacterial Genomes
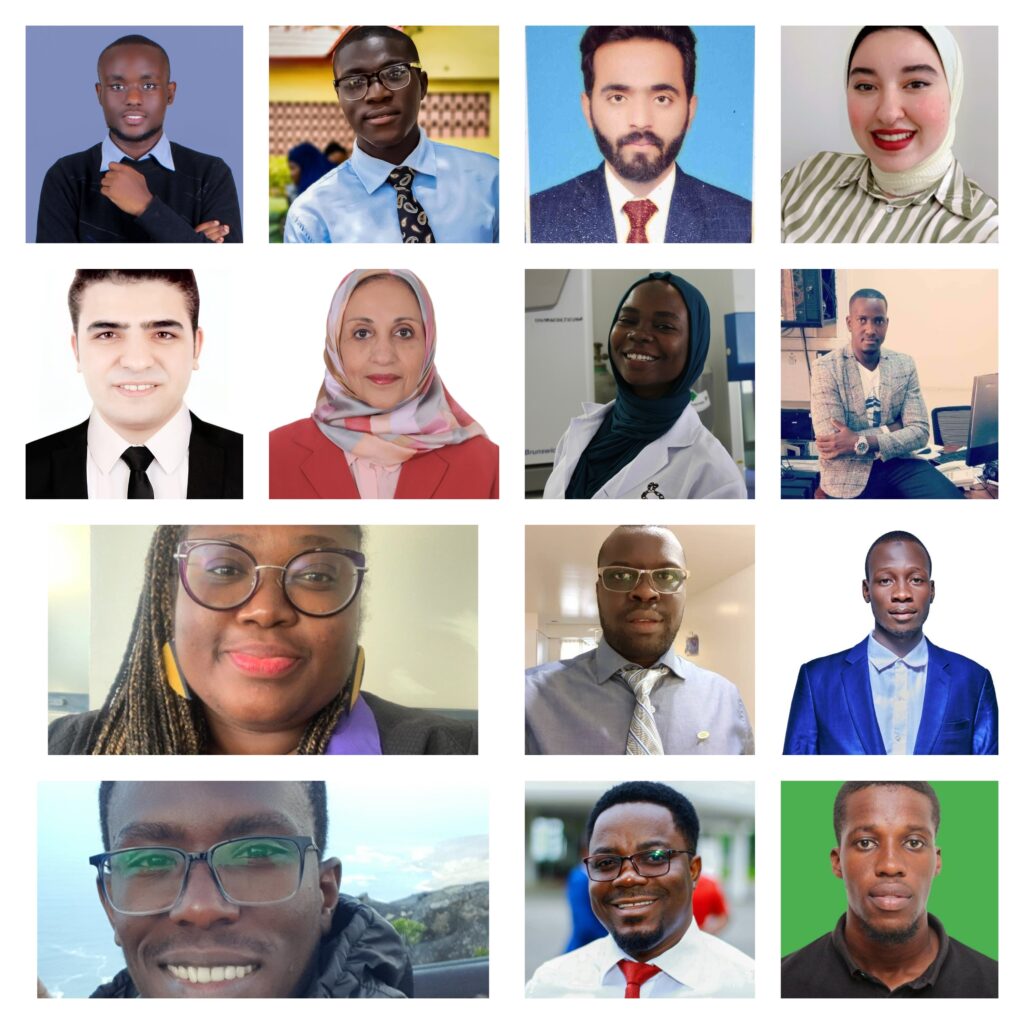
Martin Njau Kamau, Mohamed S. AboHoussein, Olawale Adejumobi, Reem Salam, Sabreen Ali Abo Al-Hassan, Shafqat Hussain, Tosin Senbadejo, Marilyne Aza-Gnandji, Mamadou Coulibaly, Lindani Moyo, Kadmiel Adjetey, John Ngjogu, Gyasi Kweku Foh, Davis Kuchaka, and Abdulkadir Ibrahim
In our project, we aim to tackle the global health challenge of antimicrobial resistance (AMR) by developing an advanced bioinformatics pipeline. In this study, we designed and implemented Bacgen as a tool to automate the process of detection and analysis of antimicrobial resistance genes, plasmids, and virulence factors from bacterial whole-genome sequences. It will use discovered AMR genes, plasmids, and virulence factors as input for pattern investigation through network analysis. By integrating quality control, assembly, detection, and network analysis steps, Bacgen will provide a powerful solution for unveiling the dynamics of AMR dissemination. This project identifies important interactions and pathways in bacterial populations, thus giving valuable insights into mechanisms driving AMR. Bacgen is essential because it increases the capacity for monitoring, understanding, and combating antimicrobial resistance, thereby supporting, in the long run, the development of effective public health strategies.
Comparative Genome Analysis of Nipah Virus and SARS-CoV-2 using Bioinformatics
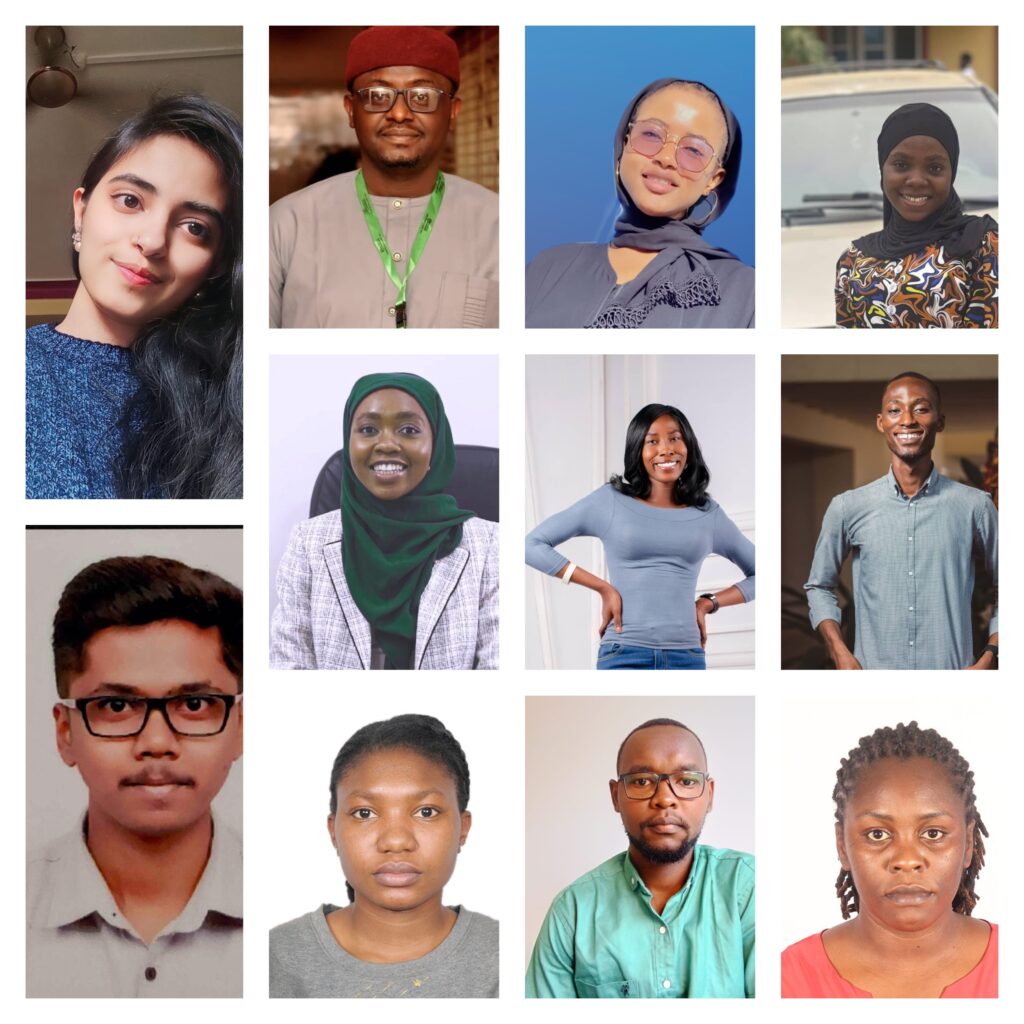
Renuka Jojare, Umar Faruk Abubakar, Zulfa Ismail Shabani, Rasheedat Ameen, Raj Gondane, Purity Oreng’, Peter Kimani Muchina, Patricia Oyeronke Ogundare, Kaothar Owolabi, Emmanuel Adu Sarpong and Doreen Kinya
This project delves into the genomic analysis of SARS-CoV-2 (the virus that causes COVID-19) and the Nipah Virus using advanced deep learning techniques with Keras and TensorFlow.
In this study, we trained a neural network model to effectively classify the genetic sequences of these viruses. By encoding the sequences into a numerical format and employing a confusion matrix for evaluation, we strive to achieve accurate differentiation between the two viruses. This approach not only enhances our understanding of their genetic structures but also aids in tracking mutations and informing public health strategies.
In-silico Prediction of Small Molecules as Potential TGR5 Agonists in Diabetes Treatment
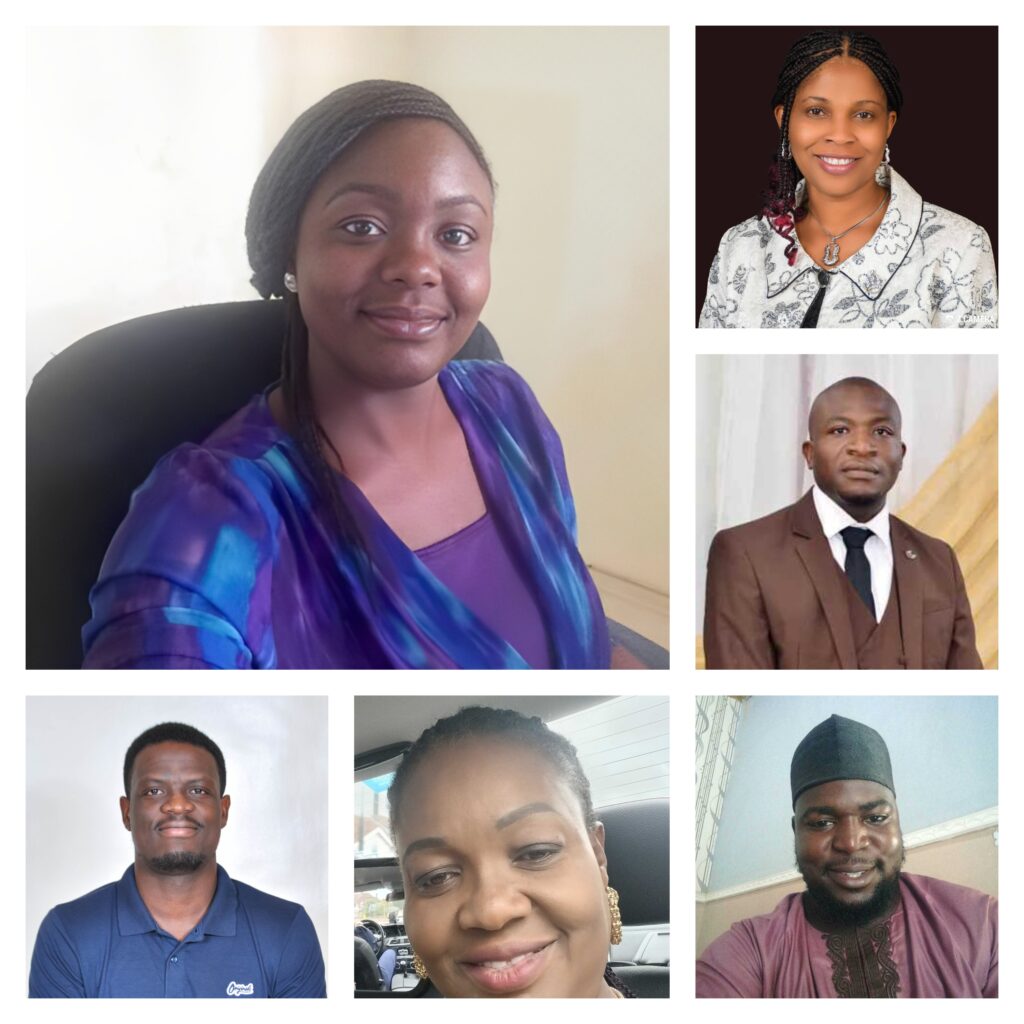
Ojochenemi A. Enejoh, Chinelo Henrietta Okonkwo, Kemiki Olalekan, Hector Nortey, Florence Mbaoji and Abdulrasak Sale
Diabetes is a chronic metabolic disorder, marked by elevated blood glucose levels, which can lead to sever complications. One current research approach to the treatment of diabetes is exploring the role of the TGR5/GLP1 pathway in improving glucose metabolism. TGR5’s main function is to regulate blood glucose levels and increase energy expenditure, making it a promising candidate for treating type 2 diabetes. TGR5 agonists have now emerged as a promising class of therapeutic agents for the treatment of type 2 diabetes.
In this study, we predicted novel small molecules as possible TGR5 agonists in the treatment of diabetes, aided by the use of machine learning-based models, molecular docking simulation, and molecular dynamics simulation techniques. The progress made in machine learning has not only enhanced our comprehension of diabetes but also created avenues for the creation of innovative therapies, which can be used to identify small molecules that can act as TGR5 agonists.
Influence of Natural Diet on the Metabolomic Profile of Drosophila melanogaster

Oluwatobi Fijabi, Vincent Duru, Abdulazaeez Giwa and Angela Krobea
In this study, we analyzed untargeted metabolomics data from peach and strawberry manipulated diets and Drosophila larvae. Using natural variation that exists in the Drosophila DGRP lines, we intend to uncover the mechanisms that may govern metabolic syndrome in humans. Our metabolomic data were generated using the gas chromatography/mass spectrometry at the UC Davis core facility while we applied broad spectrum antibiotics and autoclaving treatments on our diet to eliminate dietary microbes. We expect genotype-by-diet interaction to modulate the metabolites compositions of larvae occasioned by dietary composition and nutrient levels. Our study reveals the importance of processing diets and the implications of microbes in the pathogenesis of metabolic disorder.
Investigating Candidate Genes Involved in Esophageal Cancer through Integrated Multi-omic Data Analysis
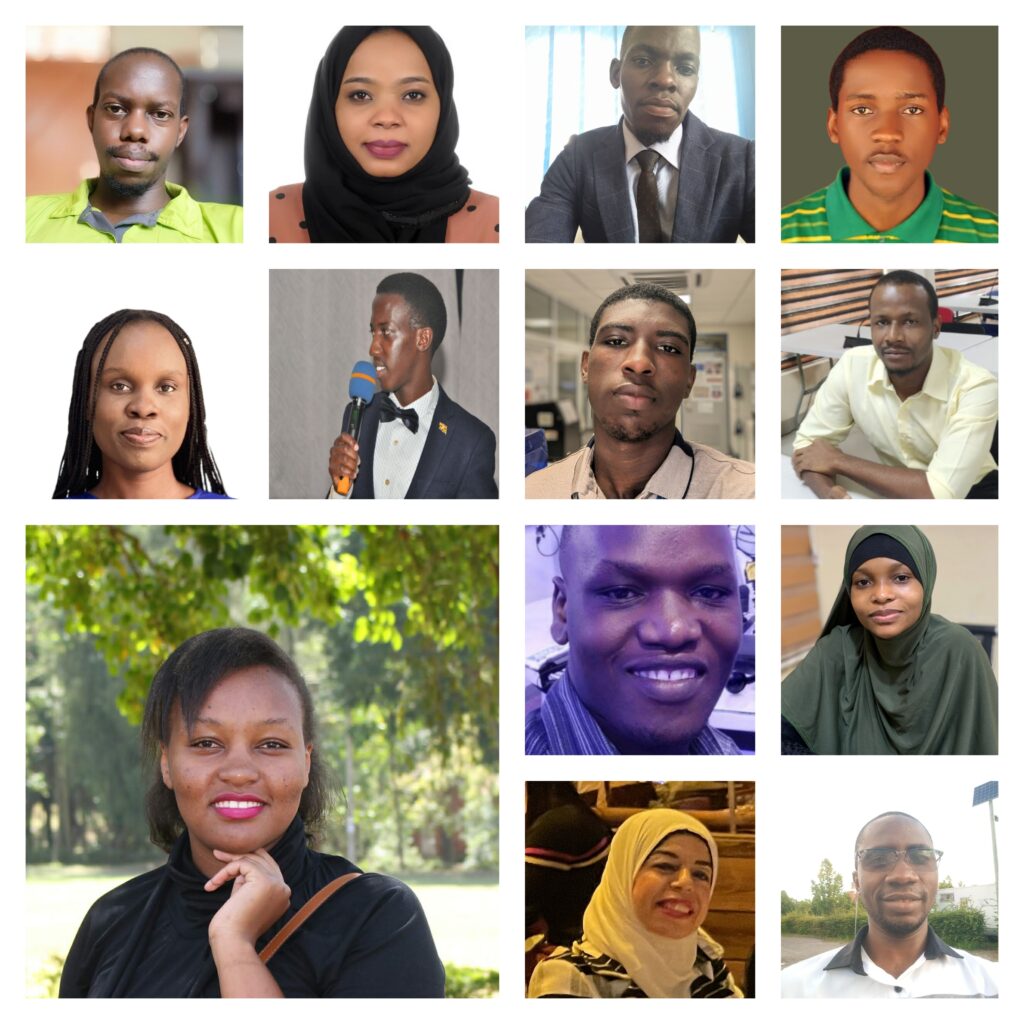
Patriciah Kinyua, Esra Alsadig Abdalwhab Abdallah, Emmanuel Aroma, Gershom A. Olajire, Faith Adegoke, Emmanuel Asiimwe, Sadock Mboya, Andrew Aladele, Kwame Boamah Buabeng, Tayyiba Amartey, Wafaa M. Rashed, Yusuf Eshimutu Abu and Mark Kivumbi
Esophageal cancer remains a significant global health challenge, with high morbidity and mortality rates. To address this, our project aims to identify and investigate candidate genes involved in esophageal cancer through a comprehensive multi-omics approach. By integrating data from genomics, transcriptomics, proteomics, and epigenomics, we will gain a deeper understanding of the molecular mechanisms driving this disease.
Our multidisciplinary team will employ cutting-edge bioinformatics and systems biology techniques to identify differentially expressed genes, proteins, and epigenetic modifications between esophageal cancer and normal tissues. We will explore the functional relationships and interactions among these molecular entities, aiming to uncover novel therapeutic targets and drug-gene interactions. We will utilize public repositories such as TCGA and GEO to gather diverse datasets encompassing various stages and subtypes of esophageal cancer.
Through this project, we hope to contribute to the development of precision medicine strategies for esophageal cancer, ultimately improving patient outcomes and advancing the field of cancer research. By pushing the boundaries of multi-omics research and leveraging the power of integrated data analysis, we strive to make significant strides in the fight against esophageal cancer, offering new hope and better treatment options for affected individuals.